The 2021 round of PODS funding strongly encourages works that transform research domains through data science and AI, works that improve the reproducibility of research, and works that promise major impact and potential for significant expansion. 17 teams receive funding support for a wide range of exciting projects with data science and Artificial Intelligence (AI) as the common thread. Researchers on these projects are from 9 schools and colleges on the Ann Arbor campus and from the Flint campus.
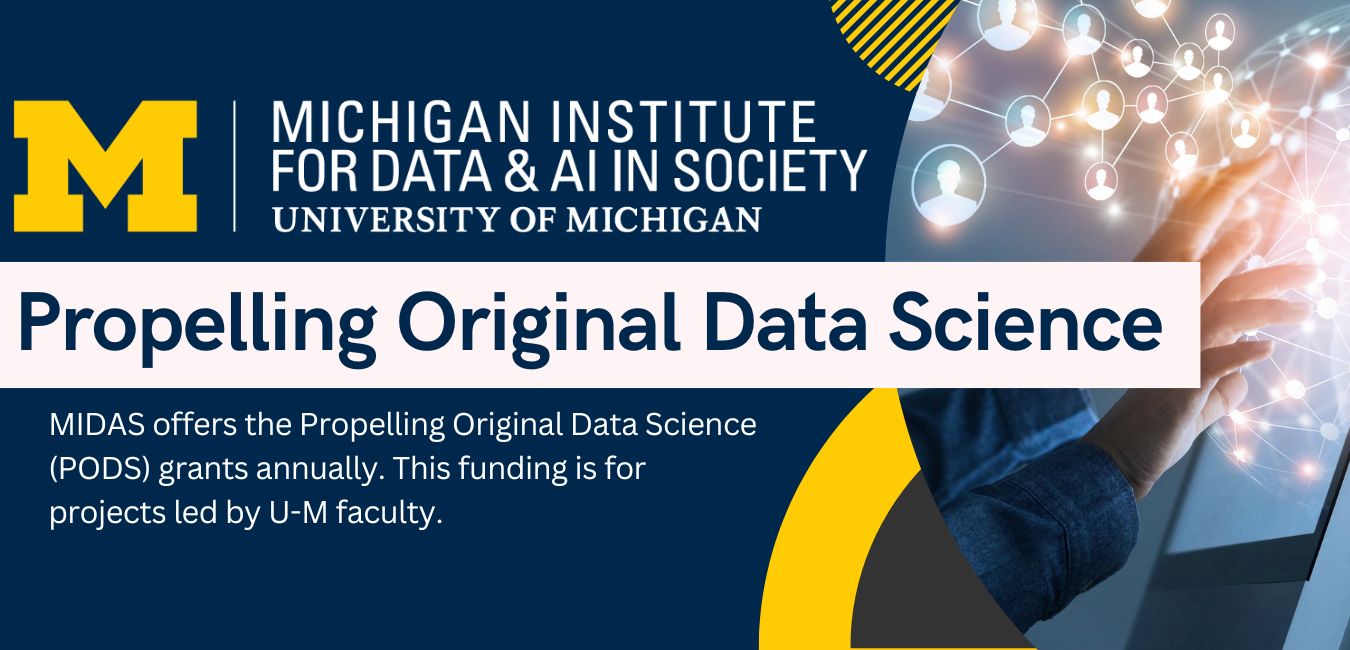
Awarded Projects
Below are the projects that were awarded PODS grants, listed with their (co) Principal Investigators.
- Images to Integrated Data: Piloting new methods to digitize, parse, and link historical records, J. Trent Alexander (Inter-university Consortium for Political and Social Research), Sara Lafia (Inter-university Consortium for Political and Social Research)
- Detecting Early-Warning Signals of Market Share Loss from Locus of Customer Movements, Syagnik Banerjee (Marketing), Halil Bisgin (Computer Science), Murali Mani (Computer Science), U-M Flint
- Coordinated Multi-building Modeling and Management for Flexible Grid Service Innovation, Eunshin Byon (Industrial and Operations Engineering), Raed Al Kontar (Industrial and Operations Engineering)
- Exploring Attention-based Deep Learning Methods for Improving Students’ Ability to Engage with Scientific Literature, Kevyn Collins-Thompson (School of Information), Yulia Sevryugina (U-M Library)
- Robust Machine Learning under Distribution Shifts and Shocks: Application to Sustainable Air Quality, Paramveer Dhillon (School of Information)
- IPODS: Innovative and Powerful Optimization Methods for Data Science with Statistical Guarantees, Salar Fattahi (Industrial and Operations Engineering), Albert Berahas (Industrial and Operations Engineering)
- Supporting Decision-making for a Vital Waterway in the Great Lakes by Machine Learning Model-based Lake Ice Forecasting, Ayumi Fujisaki-Manome (Climate and Space Sciences and Engineering, Cooperative Institute for Great Lakes Research), Christiane Jablonowski (Climate and Space Sciences and Engineering)
- Interpretable Machine Learning for Identifying Descriptors of Catalysts for Chemical Conversion, Bryan Goldsmith (Chemical Engineering), Suljo Linic (Chemical Engineering)
- Ensuring FAIRness in Social Media Archives, Libby Hemphill (Inter-university Consortium for Political and Social Research)
- Measuring Racial Disparity in the Language of Physician-Patient Interactions, David Jurgens (School of Information), Allison Earl (Psychology)
- Equitable Models for Persistent Opioid Use Prediction and Personalization, Rahul Ladhania (Health Management and Policy), Anne Fernandez (Psychiatry)
- Discovering Causes of Cancer Recurrence Through Inverse Reinforcement Learning, Gary Luker (Radiology), Jennifer Linderman (Chemical Engineering), Kathryn Luker (Radiology)
- Using Geospatial Data Science to Identify Vulnerable Communities to Climate Change, Joshua Newell (School for Environment and Sustainability), Marie O’Neill (Environmental Health Sciences), Carina Gronlund (Survey Research Center)
- Machine Learning Augmented System for Continuous Fetal Monitoring, Kathleen Sienko (Mechanical Engineering), Carrie Bell (Obstetrics and Gynecology), Noel Perkins (Mechanical Engineering)
- Classifying the Content of Undergraduate Course-taking at Scale, Kevin Stange (Public Policy), Allyson Flaster (Inter-university Consortium for Political and Social Research)
- Data Science Approach towards a Socio-ecological Framework for the Investigation of Continental Urban Stream Water Quality Pattern, Runzi Wang (School for Environment and Sustainability), Yang Chen (Statistics), William S. Currie (School for Environment and Sustainability)
- Scientifically-Structured Latent Variable Methods for High-Dimensional Data to Individualize Healthcare, Zhenke Wu (Biostatistics)