Overview
The annual Summit (previously known as the annual Symposium) is the largest annual data science and AI event on campus. The event brings together the U-M data science and AI research community and their external collaborators to build research vision and collaboration. It also showcases the breadth and depth of U-M data science and AI research, from theory and methodology development to the transformative use of data and AI to address scientific and societal challenges in all domains. The event is free for all attendees (U-M faculty, staff, and trainees, as well as industry, government and community members).
Schedule
Dr. H. V. Jagadish, Edgar F Codd Distinguished University Professor and Bernard A Galler Collegiate Professor of Computer Science and Engineering; MIDAS Director
Opening Remarks Recording Opening Remarks Slides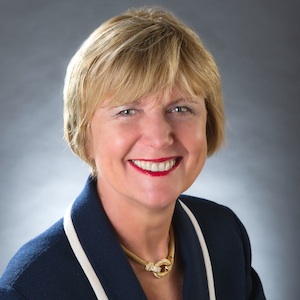
Dr. Suzanne Bakken
Professor of Biomedical Informatics and Alumni Professor of the School of Nursing, Columbia University; Editor-in-Chief, Journal of the American Medical Informatics Association
Dissemination is key to advancing discovery and application in health data science. Dr. Bakken will share her perspective as Editor-in-Chief of the Journal of the American Medical Informatics Association on the opportunities for disseminating health data science and key attributes for success.
This new campus-wide program will train 60 postdocs in the next six years and, with the postdoc program at the core, build momentum for the campus research community and external collaborators to use AI methods for breakthroughs in science and engineering research.
AI Science Talks
“* ” Detonates presentation has a recording and/or slides available for download
Dr. H. V. Jagadish, Edgar F Codd Distinguished University Professor and Bernard A Galler Collegiate Professor of Computer Science and Engineering; MIDAS Director
Dr. H. V. Jagadish will introduce the audience to this exciting new postdoctoral program and set the stage the upcoming talks by distinguished U-M researchers.
Atul Prakash, Associate Chair, Division of Computer Science and Engineering, Department of Electrical Engineering and Computer Science and Professor of Electrical Engineering and Computer Science, College of Engineering
Deep Neural networks (DNN) are known to be vulnerable to adversarial inputs. We describe some recent results towards building more robust DNN classifiers in two settings: (1) physical perturbation attacks on real-world objects and (2) deepfake detection. On (1), we describe a system called GRAPHITE that automatically and efficiently generates candidates for robust physical perturbation attacks on hard-label blackbox classifiers. Our hope is that GRAPHITE can help lead to advances in defense against robust physical perturbation attacks, which remains an open problem. On (2), we address a significant challenge that adversarial perturbations can be misused by deepfake designers to overcome state-of-the-art deepfake detectors.
Yang Chen, Assistant Professor of Statistics, College of Literature, Science, and the Arts
In this talk, I will briefly summarize a few data driven approaches that we have developed for space weather forecasting, including techniques for solar flare predictions and quantified terrestrial impacts of major space weather events.
Michael Meyer, Professor of Astronomy, College of Literature, Science, and the Arts
Artificial Intelligence has been applied in several ways to make progress in understanding planets around other stars, placing our Solar system, and the potential for life that it represents, in context within our Milky Way galaxy: a) advanced image processing to remove light from the central star to find planets; b) efficient modeling of planet spectra in high dimension spaces; and c) discerning subtle correlations between planet properties in several dimensions in order to build predictive theories of formation and evolution.
Jacinta Beehner, Professor of Psychology and Professor of Anthropology, College of Literature, Science, and the Arts
Thore Bergman, Professor of Psychology and Professor of Ecology and Evolutionary Biology, College of Literature, Science, and the Arts
Animal movements are key to understanding many biological phenomena. The largest movement dataset from primates derives from 25 collared baboons in Kenya across a two-week timeframe (a dataset that single-handedly produced two Science papers and a handful of other publications). However, deploying on-animal-sensors in primates is extremely difficult and even where it can be done, it may not be ethical if it puts animals at risk. We envision a landscape that uses AI to do the heavy-lifting for us. We propose to outfit a forest (not the animals) with the ability to monitor the movement of individual primates. We have a small, tractable forest in Costa Rica where we can track animals using a grid of acoustic sensors placed throughout the landscape. Because primate vocalizations have individual signatures, deep learning can be harnessed to identify individual monkeys as they traverse across the landscape. The potential for understanding the social dynamics within and across animal groups in their natural habitats would be unparalleled.
Alex Gorodetsky, Assistant Professor of Aerospace Engineering, College of Engineering
Complex and computationally expensive simulations are increasingly used to probe complex systems that are inaccessible through experimental approaches. However, these simulation tools are rife with uncertainty: unknown parameters, unknown initial conditions, model errors — these all contribute to significant risk for using simulations for important decisions. In this lighting talk we discuss emerging techniques for computationally enabling uncertainty quantification at scale.
Kira Barton, Associate Professor of Robotics and Associate Professor of Mechanical Engineering, College of Engineering
Dawn Tilbury, Associate Vice President for Research-Convergence Science, University of Michigan Office of Research, Ronald D and Regina C McNeil Department Chair of Robotics, Herrick Professor of Engineering, Professor of Robotics, Professor or Mechanical Engineering and Professor of Electrical Engineering and Computer Science, College of Engineering
Rapid advances in artificial intelligence (AI) have the potential to significantly increase the productivity, quality and profitability in manufacturing systems. Traditional mass-production will give way to personalized production, with each item made to order, at the low cost and high-quality consumers expect. Manufacturing systems will be resilient to multiple disruptions, from small-scale machine breakdowns, to large-scale natural disasters. Products will be made with higher precision and lower variability. Gains have been made towards this vision of Industry 5.0, a sustainable, resilient, and human-centric manufacturing system that uses AI as a tool. Despite early successes, challenges remain to realize this vision.
Paul Zimmerman, Professor of Chemistry, College of Literature, Science, and the Arts
Ambuj Tewari, Professor of Statistics, College of Literature, Science, and the Arts and Professor of Electrical Engineering and Computer Science, College of Engineeri
Molecular properties depend not only on chemical connectivity (bonds expressible in a graph), but also on the broad geometric space involving angles and torsions between these bonds. Sampling of this space is considered a grand challenge for computation due to the combinatorial expansion in number of conformers with size of molecule. In this talk, we present a modern reinforcement learning approach to the conformer sampling problem – which is trained via a carefully designed curriculum –and discuss the principles behind this strategy.
David Gerdes, Arthur F Thurnau Professor, Professor of Physics, Chair, Department of Physics and Professor of Astronomy, College of Literature, Science, and the Arts
How can we detect an asteroid the size of Ann Arbor at twice Neptune’s distance from the Sun—an asteroid we could potentially visit with a spacecraft? I’ll describe the DECam Ecliptic Exploration Project (DEEP), a UM-led astronomical survey intended to discover thousands of the faintest solar system objects ever detected from earth. We have developed a novel approach to moving-object detection in astronomical images that uses machine learning to reduce backgrounds by roughly a factor of one million. I’ll describe how this technique can be extended to even fainter objects by combining data from multiple nights and even multiple telescopes. In this way, we hope to discover a flyby target for NASA’s New Horizons spacecraft, which flew by the Pluto system in 2015 and is now passing through the outer regions of the Kuiper Belt.
Eunshin Byon, Associate Professor of Industrial and Operations Engineering, College of Engineering
Advances in numerical algorithms and computing power bring digital twins to the forefront of operational analysis of many systems. Typically, digital twins are developed based on physics-based first principles and require various parameters to be specified. Some of these parameters are not observable in physical systems. When physical laws to identify those parameters are unavailable, educated guesses are employed, but inappropriate assumptions can cause substantial deviations in a digital twin’s outputs from the actual system. To make digital twins represent near-exact replicas of real systems, we leverage the power of Big Data to identify those unknown parameters with observational data.
Raed Al Kontar, Assistant Professor of Industrial and Operations Engineering, College of Engineering
Judy Jin, Professor of Industrial and Operations Engineering, Professor of Integrative Systems and Design and Director Academic Program, Integrative Systems and Design, College of Engineering
Eunshin Byon, Associate Professor of Industrial and Operations Engineering, College of Engineering
The computational power at the edge device is steadily increasing. AI chips are rapidly infiltrating the market. Tesla’s autopilot system has compute power equivalent to hundreds of Macbook pros, and small local computers such as Raspberry Pis have become commonplace in manufacturing. This change opens a new paradigm of AI-driven data analytics for connected systems within IoT for cross-learning and optimal decisions. We will discuss our efforts in data analytics aimed at bringing this future into reality with applications in manufacturing and sustainable energy systems. AI techniques for transfer learning and federated uncertainty quantification and feature extraction will be highlighted.
William Currie, Professor and Associate Dean for Research and Engagement of School for Environment and Sustainability; Schmidt AI in Science Program co-Director
Dr. William Currie will summarize the session’s talks and conclude the session’s presentation of the Schmidt AI in Science Program. There may also be sufficient time for a brief audience Q&A during this segment.
Showcasing a wide range of data science and AI research projects and grassroots data science and AI organizations.
2022 Poster Award Recipients
BOLD denotes the presenting author
Overall Winner
Harkirat Singh Arora: BME, University of Michigan
Sriram Chandrasekaran: BME, University of Michigan
Summary: Antibiotic resistance is becoming a significant public health concern worldwide, with few novel treatments being discovered. Drug combination therapy is a promising solution against antibiotic resistance. But, the search for effective drug combinations within a vast combinatorial space is time- and resource-intensive. We developed an ML-based algorithm that, (1) predicts effective multi-drug therapies, (2) utilizes multi-omics datasets for uncovering complex drug-drug interactions, (3) overcomes the need for high-cost experimental datasets, (4) provides the lucid interpretation of model predictions, and (5) accounts for drug toxicity profiles to design safer treatments. Current approaches cannot address more than one of the above concerns simultaneously.
Methods: The approach involves a three-step process, (1) generating feature profiles for individual drug treatments using multi-omics data such as metabolomics, proteomics, structural profiles, etc., followed by (2) preprocessing to compute joint profiles for a combination accounting for similarity and uniqueness among drugs in the treatment, and (3) feeding the information to the ML algorithm for model development, and evaluating performance.
Results: Performance for the approach was evaluated on several drug combination datasets, two-way interactions (R=0.6015***), two-way interactions in Glycerol (R=0.5178***), three-way interactions (R=0.4515***), sequential interactions (R=0.4337***) [*** indicates p-value < 1e-3]. The trained model was interpreted using the Testing with Activation Concept Vectors (TCAV) approach, which concludes that subsystems like Pyruvate metabolism, TCA cycle, and Oxidative Phosphorylation play an important role in predictions made by the model. Additionally, the trained model was fine-tuned to predict the toxicity of combination therapy to ensure the safety of the treatment.
Impact: As novel treatments are not readily discovered, it makes it crucial to design treatments using approved FDA drugs. Our developed algorithm aims to provide an innovative and unique perspective on utilizing machine learning in guiding the development of multi-drug treatments using FDA drugs.
Promising Language-Oriented Research
Eric Martell: Psychology, University of Michigan
Natalie Robbins: Cognitive Science, Linguistics, and Romance Languages and Literature, University of Michigan
Natasha Vernooij: Psychology, University of Michigan
Logan Walls: Psychology, University of Michigan
While there are sources for Spanish-English bilingual speech data, they are not sufficiently accessible for analysis. We propose an open-access data repository of Spanish-English bilingual speech called ES COCO (English-Spanish COde-switching COrpus). ES COCO will contain tagged speech from podcasts and already-created corpora, and meta-data such as speaker and demographic information. While some multilingual corpora exist (ex. BilingBank (MacWhinney, 2019)), this will be the largest Spanish-English corpus where researchers do not need to aggregate data. Most of the ES COCO data are not-transcribed audio recordings.
We use the XLS-R neural network, fine-tuned on the Spanish and English components of the CommonVoice dataset, for speech-to-text conversion (Babu et al., 2021; Ardila et al., 2020). We increase the accuracy by boosting it with an n-gram language model trained on Spanish-English datasets from the LinCE Benchmark (Aguilar et al., 2020). Once converted to text, we apply an automated tagging process for part-of-speech and language to annotate linguistic features of the data. These processes rely on a transformer language model, XLM RoBERTa (Conneau et al., 2019), fine-tuned using Spanish-English datasets from LinCE (Aguilar et al., 2020).
In addition to providing the corpus in a machine-readable format, we enable data exploration with a user-friendly interface, which can be run locally on the user’s machine or accessed via web browser. Users can search and filter the corpus by linguistic feature and view results in context, allowing them to quickly answer questions about bilingual language practices.
By creating this corpus of Spanish-English speech data, we remove the largest barriers in language research: the time and financial cost of collecting, transcribing, and tagging data. ES COCO is particularly beneficial for researchers who are not at R1 institutions and have limited access to funding, personnel, time, and the language communities required for language research.
Innovative Use of Data
Kais Riani: CIS, University of Michigan
Salem Sharak: CIS, University of Michigan
Kapotaksha Das: CIS, University of Michigan
Mohamed Abouelenien: CIS, University of Michigan
Mihai Burzo: CIS, University of Michigan
Rada Mihalcea: CIS, University of Michigan
John Elson: Ford Motor Company
Clay Maranville: Ford Motor Company
Kwaku Prakah-Asante: Ford Motor Company
Waqas Manzoor: Ford Motor Company
Autonomous vehicles represent one of the most active technologies currently being developed, with research areas addressing, among others, the modeling of the states and behavioral elements of the occupants. This paper contributes to this line of research by studying the circadian rhythm of individuals using a novel multimodal dataset of 36 subjects consisting of five information channels. These channels include visual, thermal, physiological, linguistic, and background data.
Moreover, we propose a framework to explore whether the circadian rhythm can be modeled without continuous monitoring and investigate the hypothesis that multimodal features have a greater propensity for improved performance using data points specific to certain times during the day. Our analysis shows that multimodal fusion can lead to an accuracy of up to 77% on identifying energized and enervated states of the participants. Our findings highlight the validity of our hypothesis and present a novel approach for future research.
Best Layout Visualization and Clarity of Exhibition
Taigao Ma: Physics, University of Michigan
Haozhu Wang: Amazon
Jay Guo: EECS, University of Michigan
Optical multi-layer thin films are widely used in optical and energy applications requiring photonic designs. Engineers often design such structures based on their physical intuition. However, solely relying on human experts can be time-consuming and may lead to sub-optimal designs, especially when the design space is large.
In this work, we frame the multi-layer optical design task as a sequence generation problem. Based on reinforcement learning, a deep sequence generation network is proposed for efficiently generating optical layer sequences. We train the deep sequence generation network with proximal policy optimization to generate multi-layer structures with desired properties. The proposed method is applied to two energy applications.
Our algorithm successfully discovered high-performance designs, outperforming structures designed by human experts and state-of-art algorithms. We believe our algorithm based on reinforcement learning can extend to many other multi-layer tasks and achieve high performance.
List of All Research Posters
BOLD denotes the presenting author
Jiahao Shi: IOE, University of Michigan
Albert S. Berahas: IOE, University of Michigan
Zihong Yi: CSE, University of Michigan
Baoyu Zhou: ISE, Lehigh University
We propose a stochastic method for solving equality constrained optimization problems that utilizes predictive variance reduction. Specifically, we develop a method based on the sequential quadratic programming paradigm that employs variance reduction in the gradient approximations. Under reasonable assumptions, we prove that a measure of first-order stationarity evaluated at the iterates generated by our proposed algorithm converges to zero in expectation from arbitrary starting points, for both constant and adaptive step size strategies. Finally, we demonstrate the practical performance of our proposed algorithm on constrained binary classification problems that arise in machine learning
Saghar Adler: EECS, University of Michigan
Mehrdad Moharrami: Illinois Institute for Data Science and Dynamical Systems, University of Illinois at Urbana Champaign
Vijay Subramanian: EECS, University of Michigan
To highlight difficulties in learning-based optimal control in nonlinear stochastic dynamic systems, we study admission control for a classical Erlang-B blocking system with unknown service rate. At every job arrival, a dispatcher decides to assign the job to an available server or to block it. Every served job yields a fixed reward for the dispatcher, but it also results in a cost per unit time of service.
Our goal is to design a dispatching policy that maximizes the long-term average reward for the dispatcher based on observing the arrival times and the state of the system at each arrival. Critically, the dispatcher observes neither the service times nor departure times so that reinforcement learning based approaches do not apply. Hence, we develop our learning-based dispatch scheme as a parametric learning problem a’la self-tuning adaptive control. In our problem, certainty equivalent control switches between an always admit policy (always explore) and a never admit policy (immediately terminate learning), which is distinct from the adaptive control literature. Therefore, our learning scheme judiciously uses the always admit policy so that learning doesn’t stall.
We prove that for all service rates, the proposed policy asymptotically learns to take the optimal action, and we also present finite-time regret guarantees. The extreme contrast in the certainty equivalent optimal control policies leads to difficulties in learning that show up in our regret bounds for different parameter regimes. We explore this aspect in our simulations and also follow-up sampling related questions for our continuous-time system. parameter regimes. We explore this aspect in our simulations and also follow-up sampling related questions for our continuous-time system.
Fan Lai: CSE, University of Michigan
Yinwei Dai: CS, Princeton University
Sanjay S. Singapuram: CSE, University of Michigan
Jiachen Liu: CSE, University of Michigan
Xiangfeng Zhu, CSE, University of Washington
Harsha V. Madhyastha, EECS, University of Michigan
Mosharaf Chowdhury, CSE, University of Michigan
We present FedScale, a federated learning (FL) benchmarking suite with realistic datasets and a scalable runtime to enable reproducible FL research. FedScale datasets encompass a wide range of critical FL tasks, ranging from image classification and object detection to language modeling and speech recognition. Each dataset comes with a unified evaluation protocol using real-world data splits and evaluation metrics.
To reproduce realistic FL behavior, FedScale contains a scalable and extensible runtime. It provides high-level APIs to implement FL algorithms, deploy them at scale across diverse hardware and software backends, and evaluate them at scale, all with minimal developer efforts. We combine the two to perform systematic benchmarking experiments and highlight potential opportunities for heterogeneity-aware co-optimizations in FL.
FedScale is open-source and actively maintained by contributors from different institutions at fedscale.ai. We welcome feedback and contributions from the community.
Alauddin Ahmed, CSE, University of Michigan
Although machine learning (ML) models are being used in many fields to make predictions or take decisions, often we don’t know why these models make the predictions or take the decisions they do. Such an ML model commonly known as “black-box” cannot answer why it is certain about its prediction, what accounted for the uncertainty, and how much perpetuated bias exists. The lack of accountability of ML models impedes trustworthy communication between humans and models.
The current paradigm in the ML research includes model-based interpretability (e.g., linear model) and post hoc interpretability. While model-based interpretability suffers from poor accuracy, post hoc interpretability of black box models lacks adequate descriptive accuracy.
Here we present a method of developing high accuracy interpretable machine learning models in the context of materials discovery and design. Also, we attempt to establish causal relationships between input features and target outputs. In principle, this data-driven approach can be used in other disciplines, including science, arts, engineering, and health care.
Martin Ziqiao Ma: CSE, University of Michigan
Jiayi Pan: CSE, University of Michigan
Joyce Chai: CSE, University of Michigan
Humans acquire language through sensorimotor experience with the world. The ability to connect language to their referents in the physical world (referred to as grounding) play an important role in language understanding and language learning. Such ability, although effortlessly for humans, is notoriously difficult for AI agents.
To address this limitation, we introduce a new task formulation and new metrics to emphasize grounding in word learning. Specifically, we introduce Open-Vocabulary Referential Cloze (RefCloze) to challenge vision-language systems to perform visually grounded and object-centric language modeling. We propose Masked Language DEtection TRansformer (MaskDETR), a novel and simple visually grounded language model by pre-training on image-text pairs with fine-grained word-object alignment.
Through extensive experiments, we demonstrate MaskDETR as a more coherent grounded word learner, and that learning the referential grounding between words and objects is crucial to grounded word learning and processing. We further present a comprehensive inquiry on the cognitive plausibility of such vision-language transformer as a human-like word learner. The RefCloze task formulation, the new evaluation metrics, together with our empirical findings, will provide insight for future work on grounded language acquisition.
Sagnik Ray Choudhury: Learning Health Sciences, University of Michigan
Anna Rogers: Center for Social Data Science, University of Copenhagen
Isabelle Augenstein: CS, University of Copenhagen
Two of the most fundamental challenges in Natural Language Understanding (NLU) at present are: (a) how to establish whether deep learning-based models score highly on NLU benchmarks for the `right’ reasons; and (b) to understand what those reasons would even be. We investigate the behavior of reading comprehension models with respect to two linguistic `skills’: coreference resolution and comparison.
We propose a definition for the reasoning steps expected from a system that would be `reading slowly’, and compare that with the behavior of five models of the BERT family of various sizes, observed through saliency scores and counterfactual explanations. We find that for comparison (but not coreference) the systems based on larger encoders are more likely to rely on the `right’ information, but even they struggle with generalization, suggesting that they still learn specific lexical patterns rather than the general principles of comparison.
The full paper has been accepted to COLING 2022 and is available here.
Saptarshi Roy: Statistics, University of Michigan
Sunrit Chakraborty: Statistics, University of Michigan
Ambuj Tewari: Statistics, University of Michigan
Ziwei Zhu: Statistics, University of Michigan
We consider the stochastic linear contextual bandit problem with high-dimensional features. We analyze the Thompson sampling algorithm, using special classes of sparsity-inducing priors (e.g. spike-and-slab) to model the unknown parameter, and provide a nearly optimal upper bound on the expected cumulative regret. To the best of our knowledge, this is the first work that provides theoretical guarantees of Thompson sampling in high dimensional and sparse contextual bandits.
For faster computation, we use spike-and-slab prior to model the unknown parameter and variational inference instead of MCMC to approximate the posterior distribution. Extensive simulations demonstrate improved performance of our proposed algorithm over existing ones. This encourages the use of Thompson sampling algorithm in high-dimensional bandit problems arising in many modern areas like recommendation system, personalized healthcare system, experimental design, etc.
We can benefit from researchers in application domains where bandit methodology is useful. We can also benefit from collaborations with researchers working on bandits problems in fields such as computer science, operations research, electrical engineering, etc.
Eric Martell: Psychology, University of Michigan
Natalie Robbins: Cognitive Science, Linguistics, and Romance Languages and Literature, University of Michigan
Natasha Vernooij: Psychology, University of Michigan
Logan Walls: Psychology, University of Michigan
While there are sources for Spanish-English bilingual speech data, they are not sufficiently accessible for analysis. We propose an open-access data repository of Spanish-English bilingual speech called ES COCO (English-Spanish COde-switching COrpus). ES COCO will contain tagged speech from podcasts and already-created corpora, and meta-data such as speaker and demographic information. While some multilingual corpora exist (ex. BilingBank (MacWhinney, 2019)), this will be the largest Spanish-English corpus where researchers do not need to aggregate data. Most of the ES COCO data are not-transcribed audio recordings.
We use the XLS-R neural network, fine-tuned on the Spanish and English components of the CommonVoice dataset, for speech-to-text conversion (Babu et al., 2021; Ardila et al., 2020). We increase the accuracy by boosting it with an n-gram language model trained on Spanish-English datasets from the LinCE Benchmark (Aguilar et al., 2020). Once converted to text, we apply an automated tagging process for part-of-speech and language to annotate linguistic features of the data. These processes rely on a transformer language model, XLM RoBERTa (Conneau et al., 2019), fine-tuned using Spanish-English datasets from LinCE (Aguilar et al., 2020).
In addition to providing the corpus in a machine-readable format, we enable data exploration with a user-friendly interface, which can be run locally on the user’s machine or accessed via web browser. Users can search and filter the corpus by linguistic feature and view results in context, allowing them to quickly answer questions about bilingual language practices.
By creating this corpus of Spanish-English speech data, we remove the largest barriers in language research: the time and financial cost of collecting, transcribing, and tagging data. ES COCO is particularly beneficial for researchers who are not at R1 institutions and have limited access to funding, personnel, time, and the language communities required for language research.
Yu Song: Ross School of Business, University of Michigan
Puneet Manchanda: Ross School of Business, University of Michigan
With the rapid growth of online news aggregators, the debate on whether news aggregators should pay news publishers for redistributing their content has become very salient. However, there is little understanding of the impact of carrying news on news aggregators, especially for their non-news content.
Our research fills this gap by examining the impact of news on non-news user engagement and content generation on Facebook. We leverage a natural experiment, Facebook’s Australian news shutdown, to estimate this using both an event study and a difference-in-differences analysis. We find that both user engagement and content generation of non-news content on Facebook decreased after the news shutdown. We also find that these effects were more pronounced for more influential, socially active, experienced, and verified accounts.
These results suggest positive spillover effects of news content on non-news content. A simple quantification exercise shows that the impact of carrying news is economically significant for a platform like Facebook. Our results provide timely and relevant implications for regulators and social media platforms.
Kapotaksha Das: ITS, University of Michigan – Dearborn
This is a multi-faceted approach that uses multiple tools:
- OpenShift & Jenkins for daily cron job to retrieve course info and peer review results
- Google Cloud Services – BigQuery for flexible data storage
- Vertex AI to build and deploy machine learning solutions
- PyTorch and the RoBERTa transformer architecture to perform powerful natural language processing and inferences
- Tableau to build interactive and intuitive dashboards that delivers focused insights for instructors and students
Mithun Chakraborty: EECS, University of Michigan
James Edwards: EECS, University of Michigan
Sindhu Kutty: EECS, University of Michigan
Prediction markets offer an alternative to polls and surveys for the elicitation and combination of private beliefs about uncertain events. The advantages of prediction markets include time-continuous aggregation and score-based incentives for truthful belief revelation. Traditional prediction markets aggregate point estimates of forecast variables. However, exponential family prediction markets (Abernethy et al., 2014) provide a framework for eliciting and combining entire belief distributions of forecast variables.
We study a member of this family, Gaussian markets, which combine the private Gaussian belief distributions of traders about the future realized value of some real random variable. Specifically, we implement a multi-agent simulation environment with a central Gaussian market maker and a population of Bayesian traders. Our trader population is heterogeneous, separated on two variables: informativeness, or how much information a trader privately possesses about the random variable, and budget.
We draw inspiration from a previous work (Martin et al., 2021) which studied another member of the exponential family in simulation. We generalize their notion of informativeness and provide a characterization of the corresponding budget-constrained optimization process. Within our market ecosystem, we analyze the impact of trader budget and informativeness, as well as the arrival order of traders, on the market’s convergence. We also study financial properties of the market such as trader compensation and market maker loss.
Tien Nguyen: University of Michigan – Dearborn
Ryan Sutton: University of Michigan – Dearborn
Michelle Liu: University of Michigan – Dearborn
Yi-Su Chen: University of Michigan – Dearborn
The board game industry has grown tremendously in the past few decades. Partly due to the Covid-19 pandemic, its growth continues as people spent more time at home and is projected to reach revenues of around 30 billion by 2026. As another piece of evidence, the global board games market is projected to grow by $3.02 billion during the 2021-2026 with an approximate 13% of CAGR (compounded annual growth rate) and is expected to reach $13 billion by 2026 (Businesswire 2021).
On the other hand, supply chain disruptions such as cargo shortage resultant from the Covid-19 pandemic had brought about difficulty to some game publishers such as Tasty Minstrel Games that were reportedly in “virtual bankruptcy,” despite their games being largely appreciated by customers. Still others were merged with larger publishers.
In this study, we focus on the Asmodee Group, one of the major players in the board game industry, who “quietly built a board-game empire with Catan, Pandemic, and Ticket to Ride (Tullis, 2021)” through multiple merge and acquisition of smaller publishers and game distributors. Using the data from www.boardgamegeek.com (BGG), the largest online community of board-game users and designers, via Application Programming Interface (API) with Python, we obtained data in August 2021.
After data cleaning, we investigate the relationships between designer teams and game performance. We measure game performance in three different ways: including popularity of a game, ratings from the customers, and attention received from the market. Our findings provide insights on what constitutes a good design team to make a good game.
Margaret M. Reuter: BME, Michigan Medicine
Jenni Liu: BME, University of Michigan
Harkirat Singh Arora: BME, University of Michigan
Rudy J. Richardson: School of Public Health, Michigan Medicine
Sriram Chandrasekaran: BME, Michigan Medicine
Pathogens are becoming increasingly drug resistant, yet drug discovery methods have failed to produce new classes of antimicrobials for decades, thus there is an urgent need to identify effective therapies from existing FDA approved drugs. Multi-drug regimens are currently used to fight antibiotic resistance, but they are often chosen empirically, leading to suboptimal treatment outcomes and the spread of resistance.
To create new multi-drug treatment plans, computational tools are needed to narrow the vast sample size of FDA approved drugs in combination. Current computational methods rely on costly experimental data and black-box algorithms. Our model replaces omics data with drug-protein binding affinity calculations to predict effective drug combinations for Escherichia coli. Initial performance assessments show our model performs as well as models that require omics inputs. Molecular docking and neural networks were used to calculate an affinity between 59 drugs and 1499 proteins in E. coli. These drug-protein interactions were then used as features in the ML model.
During model construction the biochemical principles behind drug mechanisms of action were investigated by examining the extensive set of drug-protein interaction calculations as well as three omics studies covering chemogenomics, transcriptomics, and metabolomics. We have optimized a complex system of molecular-scale, protein-drug interactions with macro-scale, drug-drug interactions data to quickly predict drug therapies that could be used to treat deadly drug resistance pathogens.
Due to the flexible, multiscale, and hybrid nature of our model, many combinations, infeasible to interrogate via physical experiments due to cost and time, could be examined. Secondly, the model enables exploration of the underlying biological and chemical factors that influence drug mechanisms of action for better design of drug combination therapy. Our predictive model combines machine learning, deep learning, and physics-based molecular docking, which could impact and inspire future hybrid methodologies in AI and Data Science.
Jaie Woodard: Bioinformatics. University of Michigan
Chengxin Zhang: Bioinformatics. University of Michigan
Sumaiya Iqbal: Broad Institute of MIT and Harvard
Jorden Thompson: Bioinformatics. University of Michigan
Sriram Chandrasekaran: BME, University of Michigan
Alireza Mashaghi: Biophysics, Leiden University
Yang Zhang: NERS, University of Michigan
Changes resulting from a change in a single amino acid in a protein can be either disease causing or benign, due in large part to protein stability and protein binding with other proteins, nucleic acids, or small molecule ligands. We developed a database, the Annotated Database of Disease RElated Structures and Sequences (ADDRESS), mapping human genetic mutations to protein structures in the Protein Data Bank (PDB).
We found that mutations that shift the equilibrium more towards the unfolded (non-native) state are more often disease causing on average than those that approximately retain the stability. Interestingly, the threshold at which mutations become pathogenic is substantially less than the average stability of proteins in general, perhaps indicating the importance of cellular kinetics in a system where proteins are constantly degraded and misfolded.
We built decision trees inclusive of various topology relations and found that the cross relation was especially indicative of whether the mutation causes disease, in the case of non-essential proteins with low stability change. We also found that, in the case of treatability of a set of lysosomal storage disorders, stability change, binding to ligand, and an aspect of topology likely related to kinetics of the system were important in indicating whether a drug was effective. Incorporation of binding and aggregation propensity will build upon the current database.
Joshua Pickard: Department of Computational Medicine and Bioinformatics, University of Michigan
Chromatin architecture, a key regulator of gene expression, can be inferred using chromatin contact data from chromosome conformation capture or Hi-C technology. However, classical Hi-C does not preserve multi-way contacts. Here we use long sequencing reads to map genome-wide multi-way contacts and investigate higher order chromatin organization in the human genome. Multiway chromatin contact data captured with Pore-C technology contains structural information beyond the pairwise data captured with traditional Hi-C. This allows for more precise representation of chromatin architecture and lends itself to efficient representation by hypergraphs to capture this higher order network structure.
We use hypergraph theory for data representation and analysis, and quantify higher order structures in neonatal fibroblasts, biopsied adult fibroblasts, and B lymphocytes. Hypergraphs and tensors are natural representations of the contact structure in the genome.
Furthermore, we investigated the relationship between the multiway and pairwise data captured with Pore-C and Hi-C technology. By integrating multi-way contacts with chromatin accessibility, gene expression, and transcription factor binding, we introduce a data-driven method to identify cell type-specific transcription clusters. We provide transcription factor-mediated functional building blocks for cell identity that serve as a global signature for cell types.
Gabrielle Dotson, Can Chen, Stephen Lindsly, Anthony Cicalo, Sam Dilworth, Charles Ryan, Sivakumar Jeyarajan, Walter Meixner, Cooper Stansbury, Joshua Pickard, Nicholas Beckloff, Amit Surana, Max Wicha, Lindsey Muir, and Indika Rajapakse. “Deciphering Multi-way Interactions in the Human Genome.” Nature Communications, in Press (2022).
Joshua Pickard, Rahmey Salhm, Can Chen, Amit Surana, Indika Rajapakse. “Hypergraph Analysis Toolbox for Long Read Sequencing,” Manuscript in preparation
Kais Riani: CIS, University of Michigan
Salem Sharak: CIS, University of Michigan
Kapotaksha Das: CIS, University of Michigan
Mohamed Abouelenien: CIS, University of Michigan
Mihai Burzo: CIS, University of Michigan
Rada Mihalcea: CIS, University of Michigan
John Elson: Ford Motor Company
Clay Maranville: Ford Motor Company
Kwaku Prakah-Asante: Ford Motor Company
Waqas Manzoor: Ford Motor Company
Autonomous vehicles represent one of the most active technologies currently being developed, with research areas addressing, among others, the modeling of the states and behavioral elements of the occupants. This paper contributes to this line of research by studying the circadian rhythm of individuals using a novel multimodal dataset of 36 subjects consisting of five information channels. These channels include visual, thermal, physiological, linguistic, and background data.
Moreover, we propose a framework to explore whether the circadian rhythm can be modeled without continuous monitoring and investigate the hypothesis that multimodal features have a greater propensity for improved performance using data points specific to certain times during the day. Our analysis shows that multimodal fusion can lead to an accuracy of up to 77% on identifying energized and enervated states of the participants. Our findings highlight the validity of our hypothesis and present a novel approach for future research.
Harkirat Singh Arora: BME, University of Michigan
Sriram Chandrasekaran: BME, University of Michigan
Summary: Antibiotic resistance is becoming a significant public health concern worldwide, with few novel treatments being discovered. Drug combination therapy is a promising solution against antibiotic resistance. But, the search for effective drug combinations within a vast combinatorial space is time- and resource-intensive. We developed an ML-based algorithm that, (1) predicts effective multi-drug therapies, (2) utilizes multi-omics datasets for uncovering complex drug-drug interactions, (3) overcomes the need for high-cost experimental datasets, (4) provides the lucid interpretation of model predictions, and (5) accounts for drug toxicity profiles to design safer treatments. Current approaches cannot address more than one of the above concerns simultaneously.
Methods: The approach involves a three-step process, (1) generating feature profiles for individual drug treatments using multi-omics data such as metabolomics, proteomics, structural profiles, etc., followed by (2) preprocessing to compute joint profiles for a combination accounting for similarity and uniqueness among drugs in the treatment, and (3) feeding the information to the ML algorithm for model development, and evaluating performance.
Results: Performance for the approach was evaluated on several drug combination datasets, two-way interactions (R=0.6015***), two-way interactions in Glycerol (R=0.5178***), three-way interactions (R=0.4515***), sequential interactions (R=0.4337***) [*** indicates p-value < 1e-3]. The trained model was interpreted using the Testing with Activation Concept Vectors (TCAV) approach, which concludes that subsystems like Pyruvate metabolism, TCA cycle, and Oxidative Phosphorylation play an important role in predictions made by the model. Additionally, the trained model was fine-tuned to predict the toxicity of combination therapy to ensure the safety of the treatment.
Impact: As novel treatments are not readily discovered, it makes it crucial to design treatments using approved FDA drugs. Our developed algorithm aims to provide an innovative and unique perspective on utilizing machine learning in guiding the development of multi-drug treatments using FDA drugs.
Corwin Kerr: Chemical Engineering, University of Michigan
Shih-Kuang Lee: Materials Science & Engineering, University of Michigan
Brandon Butler: Chemical Engineering, University of Michigan
Sharon Glotzer: Chemical Engineering, Materials Science & Engineering, and Biointerfaces Institute, University of Michigan
Managing file-based workflows is a cross-disciplinary headache. We highlight how tools originally developed to manage simulation data can be applied to simplify certain tasks in machine learning and data science, like generating data, selecting models, and streamlining the hyperparameter optimization of neural networks. The signac framework consists of three Python packages to help organize file-based projects, define reproducible computational workflows, and explore the data. It provides a command line and Python interface to access and manage project data as well as submit cluster jobs to high performance computing schedulers.
Signac implements a file-based database with no need to explicitly define a data schema. Signac organizes collections of parameter values as signac jobs and stores them in a flat directory structure. Using the command line or Python query interface, you can access data stored in the job directory, get job-specific file paths, and generate human-readable directory structures for sharing. This frees you from thinking about the minutiae of file organization and lets the data schema evolve with the project.
Signac-flow lets you define a computational workflow composed of operations with pre- and post-conditions. Using the command line interface, operations can be run locally or submitted to high performance computing systems and features built-in support for GreatLakes. The signac-dashboard package helps you inspect and filter jobs in a signac project. It runs a local web server and can interactively display files, videos, and images such as learning curves.
Developers and users are active in the Slack channel and happy to welcome new users. Check out signac.io for more.
Taigao Ma: Physics, University of Michigan
Haozhu Wang: Amazon
Jay Guo: EECS, University of Michigan
Optical multi-layer thin films are widely used in optical and energy applications requiring photonic designs. Engineers often design such structures based on their physical intuition. However, solely relying on human experts can be time-consuming and may lead to sub-optimal designs, especially when the design space is large.
In this work, we frame the multi-layer optical design task as a sequence generation problem. Based on reinforcement learning, a deep sequence generation network is proposed for efficiently generating optical layer sequences. We train the deep sequence generation network with proximal policy optimization to generate multi-layer structures with desired properties. The proposed method is applied to two energy applications.
Our algorithm successfully discovered high-performance designs, outperforming structures designed by human experts and state-of-art algorithms. We believe our algorithm based on reinforcement learning can extend to many other multi-layer tasks and achieve high performance.
Nathanial Lydick: Physics, University of Michigan
Lingxiao Zhou: Physics, University of Michigan
Rahul Gogna: KLA Corporation
Hui Deng: Physics, University of Michigan
Moiré patterns in van der Waals heterostructures have lately received a significant focus in 2D materials research. They are open to external control through the twist-angle between the layers while providing significant impact on the band-structure and properties of the heterostructure, such as “magic-angle” superconducting graphene. While existing nanoscale measurement techniques such as transmission electron microscopy and near-field tip-enhanced microscopy are able to directly measure the Moiré patterns, these techniques are typically slow, costly, and often require sample preparation incompatible with other measurements and experiments.
We attempt to overcome these limitations by applying machine learning to the far-field data scattered through a metalens placed in the near-field region of the sample. The metalens, which consists of a collection of dipole resonators placed in the near field of the sample, is able to scatter the evanescent high-spatial-frequency near-field information to detectors in the far field. Using a U-Net convolutional neural network trained according to the metalens scatterer arrangement, we are able to reconstruct the near-field pattern from the scattered far-field data.
We model the problem using a simulation of the metalens that models the interaction of the resonant dipoles with the near-field as well as the dipole-dipole interactions within the metalens. This allows us to quickly generate a training dataset of tens of thousands of near-field configurations and far-field output. Using this, we investigate the effect of different metalens designs on the near-field reconstruction.
These results pave the way for future physical implementations to allow direct single-shot measurement of Moiré lattices in heterostructures in the far-field. They may find further application in nanofabrication metrology, enabling single-shot optical measurement of subwavelength features beyond current scanning optical techniques or electron microscopy.
Jayden Elliott: Chemical Engineering and CS, University of Michigan
Bryan R. Goldsmith: Chemical Engineering, University of Michigan
Alauddin Ahmed: ME, University of Michigan
Metal-organic frameworks (MOFs) are the pioneering candidates for solving some of the grand challenges of our society, including clean energy, carbon dioxide capture, and water purification. The crystalline nanoporous structure of these materials is advantageous for such applications compared to other solid-state materials.
However, the stability (i.e., structural integrity) of many MOFs is compromised under different operating conditions (e.g., temperature, pressure, chemical environment). Often, stability information of MOFs under these conditions is unavailable. Determining the stability of MOFs at different physicochemical conditions is a tedious experimental exercise involving multiple characterization methods. Experimentally examining the stability of MOFs under various operating conditions is impractical for the over 100,000 already-synthesized MOFs, and a standardized computational approach to determine MOFs’ stability is not available.
Here we report a comprehensive data-driven approach to predict the thermal, chemical, and mechanical stabilities of MOFs. We combine cheminformatics and materials informatics feature engineering approaches for training machine learning (ML) models. We develop four optimized ML models for the prediction of thermal, mechanical, and solvent removal stability of MOFs. The predictive performance of our ML models for thermal and solvent removal stability are better than those reported elsewhere. In principle, our models can be used for the prediction of stability of an arbitrary MOF under different operating conditions.
Taigao Ma: Physics, University of Michigan
Haozhu Wang: Amazon
Jay Guo: EECS, University of Michigan
Designing optical structures for generating structural colors is challenging due to the complex relationship between the optical structures and the color perceived by human eyes. Machine learning-based approaches have been developed to expedite this design process. However, existing methods solely focus on structural parameters of the optical design, which could lead to sub-optimal color generation due to the inability to optimize the selection of materials.
To address this issue, an approach Neural Particle Swarm Optimization is proposed. The proposed methods combine the mixture density networks as well as optimization and achieves high design accuracy and efficiency on two structural color design tasks; the first task is designing environmental-friendly alternatives to chrome coatings and the second task concerns reconstructing pictures with multilayer optical thin films. Several designs that could replace chrome coatings have been discovered; pictures with more than 200,000 pixels and thousands of unique colors can be accurately reconstructed in a few hours.
Vishnupriya Napa Ravikumar: Urban Design, University of Michigan
Cities shape people. But can people shape cities? Urban planners and designers experience a dearth of insightful scientific tools that assist them in urban research. Moreover, predominant data analysis in urban scholarship has used data to create city systems and structures that have shaped people’s access to the city and its resources – albeit inequitably.
This pattern throughout history to use data, mapping, and systematic planning to suppress the voice of the vulnerable mandates a shift in perspective. Contrary to being the “new” oil, the research argues that data has always been a resource of the powerful. Most often, data has been the key to drawing the larger pictures and connecting relationships between apparently disparate entities, resourcefully sectioned for the benefit of a few privileged groups and before the less powerful people have had a chance to get a sense for or offer reflections on the macro picture. Understandably the first known traces of data analysis and mapping were created to fight and win wars. They have been strategic attempts to achieve goals that were not always about overarching good causes.
Metricle is a counter data tool in the making that allows researchers to analyze the built landscape to retrace systemic neglect in neighborhoods from the traditional top down efforts to offer a counter-lens through which to view city design and planning. A perspective that correlates people’s needs with the existing physical landscape as opposed to performing analysis to merely establish dominion. It does this through a systematic investigation of spatial imagery in conjunction with available census and external data about the place. It uses a novel technique to co-relate and associate various related and interdependent metrics to find broad trends or anomalies in the data. These captured trends or outliers are then studied in relation to the deductions made from critical observation of spatial imagery to draw causation and speculate on recommendations.
Christopher Salisbury: ME, University of Michigan – Dearborn
Fred Feng: IMSE, University of Michigan – Dearborn
Bicycling is a promising transport mode to make our communities more sustainable, healthier, and more equitable.Motor vehicle traffic volumes, often measured by the Annual Average Daily Traffic (AADT), have been widely used in making engineering decisions. However, little data on bicycle traffic volumes have been collected and used in most U.S. cities.
In this project, we collected bicycle traffic data using a commercial automated bike counter at two locations: a multi-use path in Dearborn and a protected bike lane in Ann Arbor. Validation studies were conducted to examine the counter accuracy using video cameras. A total of 9 weeks data collection was conducted with more than 13,000 people on bikes counted as of today (the data collection in Ann Arbor is still ongoing). Data analysis was conducted to examine the bicycling traffic patterns in terms of traffic in different time of day, day of the week, and weather conditions. The primary trip purposes (i.e., commuting, recreation) at each location can be inferred from the patterns.
In addition, an open-source, public interactive dashboard (linked here) was developed that allows other researchers, traffic engineers, city planners, and the general public to freely explore the bike traffic data. The dashboard supports selecting date ranges, traffic directions, and data resolution (e.g., daily, hourly, 15-minutes). The outcome of this work can be used to get insights of bicycle infrastructure usages and support data-driven decision-making by the city planners and community engagement.
Organizational Posters
BOLD denotes the presenting author
Lucy Duan, LSA Computer Science / BCN, University of Michigan
Cristina Garbacea, EECS, University of Michigan
Jeremy Huang, Information, University of Michigan
John Kaspers, Information / Applied Data Science, University of Michigan
Ella Li, Data Science, University of Michigan
Andria Brianna Lesane, Information / Applied Data Science, University of Michigan
Jiangyue Mao, Data Science, University of Michigan
Rui Nie, Undergraduate, Statistics and Mathematics, University of Michigan
Sydney Vogel, Data Science, University of Michigan
Daniel Wang, Computer Science / Data Science, University of Michigan
The Michigan Institute for Data Science (MIDAS) Student Leadership Board is made up of 10 students representing multiple schools, programs, majors, and degree levels at the University of Michigan. The group is responsible for organizing and carrying out community service events and advising MIDAS leaders on various data science activities that benefit the student community.
Stephen Salerno: Biostatistics, University of Michigan
Soumik Purkayastha: Biostatistics, University of Michigan
In an increasingly data-driven world, data science is ubiquitous in big business and academic research. Local community organizations also stand to benefit from statistical insight; however, these groups often lack the time, resources, or skills to collect and analyze data. Statistics in the Community (STATCOM) is an outreach program that offers the expertise of graduate students, free of charge, to non-profit community and governmental organizations.
University-community partnerships such as STATCOM offer many benefits for both students and stakeholders alike. Community partners gain a deeper understanding of their operational processes and benefit from assessing program efficacy, optimizing resource allocation, and evaluating further areas of unmet need. Beyond the fulfillment of positively impacting their community, student volunteers gain hands-on experience working with data, answering complex questions, and effectively communicating statistical concepts and results to others — crucial skills of benefit throughout their careers. This poster will exemplify the unique collaboration between STATCOM at the University of Michigan and its community.
Josh Silverberg: CSE EECS, University of Michigan
Lucy Duan: LSA Computer Science / BCN, University of Michigan
Casper Guo: CSE Data Science, University of Michigan
Sachchit Kunichetty: CSE EECS, University of Michigan
Justin Paul: CSE EECS, University of Michigan
Tiffany Tan: LSA Data Science, University of Michigan
MDST (Michigan Data Science Team) is the leading practical data science and machine learning club at the University of Michigan, with over a hundred UM students working together on a range of projects each semester. We are dedicated to educating about the applications of data science and ML, while providing opportunities for members’ professional, academic, and career development. This means we work on projects, hold workshops, host corporate tech talks, and also have a couple of social events throughout the semester.
At this summit we aim to spread awareness of our club throughout the UM data science and AI community. Specifically we hope to share details of the interesting projects we work on, and the range of opportunities we offer to UM students and corporate partners alike.
Lilly Wu: CSE EECS, University of Michigan
Ashley Philip: CSE EECS, University of Michigan
Jennifer Lee: Medicine, University of Michigan
Cameron Moy: Information, University of Michigan
Tingzheng Zhou: LSA Math / Data Science, University of Michigan
Karl Godard: CSE EECS, University of Michigan
Michigan Eco Data exists to foster a community of individuals at the University of Michigan who solve environmental and biological problems with the innovative use of technology and data analysis. The organization hosts environmental-tech talks, facilitates group ecological projects, provides support to individual student’s projects, hosts group environmental trips, and plans social gatherings. Our end goal is to build and form supported network of talented students with interests in the intersection and utilization of environmentalism and data driven discovery.
Drew Bennett: Office of Research (UMOR), University of Michigan
Chris Fick: Office of Research (UMOR), University of Michigan
Innovation Partnerships experts champion the creation of corporate research alliances and collaborations to accelerate the development of promising research. We enable the translation, commercial development and licensing of groundbreaking research discoveries and technologies. We help create new ventures to usher in change.
Research Talks: Monday, November 14
Briana Mezuk, Department of Epidemiology, School of Public Health, University of Michigan
Viktoryia Kalesnikava, Department of Epidemiology, School of Public Health, University of Michigan
Linh Dang, Department of Epidemiology, School of Public Health, University of Michigan
Eskira Kahsay, Department of Epidemiology, School of Public Health, University of Michigan
Lily Johns, Department of Epidemiology, School of Public Health, University of Michigan
David Jurgens, School of Information, University of Michigan
Aparna Ananthasubramaniam, School of Information, University of Michigan
Suicide remains the 10th leading cause of death in the US. Despite decades of research, persistent gaps in understanding modifiable predictors of suicide that may inform prevention efforts remain. In response to this challenge, we aim to foster cross-disciplinary research and dialogue on suicidal behavior.
In 2003, CDC has launched the National Violent Death Reporting System (NVDRS), a comprehensive mortality surveillance system that collects salient information on suicide and other violent deaths across the US; this registry now includes over 350,000 deaths from suicide or undetermined intent. A distinct feature of the NVDRS is the inclusion of rich textual data that describe the circumstances (e.g., recent events, ongoing stressors) for most cases in the registry. These textual narratives (median character length= 545, min-max: 1-11936) are abstracted from official source documents from law enforcement and coroner/medical examiners, and contain case details that are only partially captured by other variables in the registry.
In this talk, we will discuss our ongoing research with the NVDRS data, which aims to 1) leverage narrative texts using data science and natural language processing tools, 2) identify novel suicide-related contextual features on a population scale, and 3) investigate how salient life transitions (i.e., changes in employment, relationships, housing, etc.) may relate to suicide at various life stages. We will present findings that speak to each of these elements and share encountered methodological challenges around narrative sparseness and systematic variation in the narrative length (e.g., by age, sex, educational attainment, and race of the decedent). The overall goal of this talk is to foster research dialogue and partnerships around best practices for applying emergent data science tools to identify novel correlates of suicide risk in a manner that accounts for potential biases in data collection and measurement in an equitable manner.
Rahul Ladhania, School of Public Health, University of Michigan
Lyle Ungar, University of Pennsylvania
Wenbo Wu, New York University
Nina Mazar, Boston University
Behavioral science offers some inexpensive, scalable strategies that can increase vaccination, yet most studies focus on identifying interventions which, on average, have the highest treatment effects. Without meaningful consideration of heterogeneity in treatment effects, however, there is risk of finding policies that perpetuate or exacerbate disparities. Recent developments in the field of machine learning and econometrics have brought data-driven heterogeneity estimation and personalization to the forefront. How effective is data-driven personalization of behavioral text messaging interventions to increase flu shot uptake and, from a health equity perspective, what role, if any, does race and racial bias play?
We use data from two mega-studies (Milkman et al. 2021, 2022) in three different settings (Walmart Pharmacy, a large multinational retail corporation with over 4,700 pharmacy locations across the US, with ~680,000 participants; The University of Pennsylvania and Geisinger Health Systems, two large health systems in the Northeastern United States, with ~50,000 participants), which tested the efficacy of an array of text messaging nudges encouraging actual flu shot uptake. First, we find that ML-driven personalization can make a substantial difference (upto 3X) in the effectiveness of behavioral messaging interventions for increasing flu shot uptake, over assigning all participants to the on-average best performing arms. Second, we find that gains from personalization in both settings are largely similar across racial groups in our setting. We are extending our models on data from other behavioral studies aimed at increasing COVID-19 vaccine uptake (Dai et al, 2021), assessing the transferability of inference across the two settings.
Y Z (Yang Zhang), Department of Nuclear Engineering and Radiological Sciences, University of Michigan
Despite the booming applications of AI/ML/DS methods in almost every field, one enduring challenge is the lack of explainability with the present approaches. Not being able to interpret the black-box computer models with human-understandable knowledge greatly hinders our trust and the deployment of them. Therefore, the development of Understandable/eXplainable/interpretable Artificial Intelligence (UAI/XAI) is considered as one of the main challenges. Physics and broader physical sciences provide established ground truths and thus can serve as testbeds for the development new UAI methods.
To stimulate discussions, I will briefly describe one example of our research, where we used algebraic geometry tools, namely Morse-Smale complex and sublevelset persistence homology, to produce human-understandable interpretations of autoencoder-learned collective variables in atomistic trajectories. The goal of this talk is to brainstorm and foster collaboration opportunities.
Mosharaf Chowdhury, Department of Computer Science and Engineering, University of Michigan
Although cloud computing has so far successfully accommodated the volume, velocity, and variety of Big Data, collecting everything into the cloud is becoming increasingly infeasible. Today, we face a new set of challenges. A growing awareness of privacy among individual users and governing bodies is forcing platform providers to restrict the variety of data we can collect. Often, we cannot transfer data to the cloud at the velocity of its generation. Many cloud users suffer from sticker shock, buyer’s remorse, or both as they try to keep up with the volume of data they must process. Making sense of data closer to its home is more appealing than ever.
In this talk, I will briefly introduce FedScale, a scalable and extensible open-source federated data science platform that we are building in Michigan to tackle these new challenges. FedScale provides high-level APIs for data scientists to implement their tasks, a modular design to customize implementations for diverse hardware and software backends, and the ease of deploying the same code at many scales. FedScale also includes a comprehensive benchmark that allows data scientists to evaluate their ideas in realistic, large-scale settings.
FedScale is available here.
Shasha Zou, Department of Climate and Space Sciences and Engineering, University of Michigan
Zihan Wang, Department of Climate and Space Sciences and Engineering, University of Michigan
Yang Chen, Department of Statistics, University of Michigan
Hu Sun, Department of Statistics, University of Michigan
There has been a growing awareness of space weather impacts on critical infrastructure in the civilian, commercial, and military sectors in recent years. To protect critical assets on the ground and in space, multiple federal agencies combined force and constructed the National Space Weather Strategy and Action Plan (NSWSAP). Understanding the underlying physical processes of space weather and improving the specification and forecast is a major objective of the space community. Ionospheric disturbance is highlighted as one of the five major space weather threats in the NSWSAP report.
In this presentation, I will talk about integrate modern ionosphere total electron content (TEC) dataset derived from multiple Global Navigation Satellite System (GNSS) and state-of-the-art machine learning (ML) algorithms to resolve outstanding fundamental questions of the specification and forecasting local and global ionospheric TEC and its variability.
Presentations
Michigan AI Lab Rada Mihalcea, Janice M Jenkins Collegiate Professor of Computer Science and Engineering and Professor of Electrical Engineering and Computer Science
E-Health and Artificial Intelligence Akbar Waljee, Professor of Internal Medicine, Medical School Henrike Florusbosch, Program Manager, Medical School
University of Michigan Software and Data Carpentries Patrick Schloss, Program Director of Microbiology and Immunology AP&A, Frederick G Novy Collegiate Professor of Microbiome Research and Professor of Microbiology and Immunology, Medical School
Michigan Institute for Computational Discovery and Engineering Krishna Garikipati, Professor of Mechanical Engineering, Center Director, Michigan Institute for Computational Discovery and Engineering Research, and Professor of Mathematics Karthik Duraisamy, Professor of Aerospace Engineering
University of Michigan Precision Health Sebastian Zoellner, John G Searle Associate Professor of Biostatistics, Professor of Biostatistics, and Professor of Psychiatry, Medical School
Consulting for Statistics, Computing and Analytics Research Kerby Shedden, Professor of Statistics, Professor of Biostatistics, and Center Director, Statistical Consultation and Research
Bold Challenges Dawn Tilbury, Associate Vice President for Research-Convergence Science, Ronald D and Regina C McNeil Department Chair of Robotics, Herrick Professor of Engineering, Professor of Robotics, Professor of Mechanical Engineering, and Professor of Electrical Engineering and Computer Science Arthur Lupia, Gerald R Ford Distinguished University Professor of Political Science, Professor of Political Science, Research Professor, Center for Political Studies, Institute for Social Research and Center Director, UMOR Office of the Vice President for Research
Center for Ethics, Society, and Computing Sophia Brueckner, Associate Professor of Art and Design, Associate Professor of Information, and Associate Professor of Digital Studies Institute
Digital Studies Institute Germaine Halegoua, John D Evans Development Professor, Associate Professor of Communication and Media, Associate Professor in the Digital Studies Institute and Director Graduate Studies, Digital Studies Institute
Science, Technology and Public Policy Program Molly Kleinman, Assistant Director, Ford School of Public Policy
Session Chair: Jing Liu (MIDAS Managing Director; Schmidt AI in Science Program co-Director)
Showcase Recording Showcase SlidesAll attendees are invited. Come and talk with U-M data science and AI organizations, researchers, and students, as well as industry and public-sector partners.
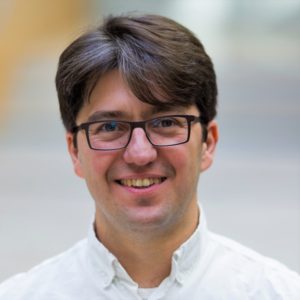
Mr. David Shor
Head of Data Science, OpenLabs R&D
We are pleased to have David Shor back to a larger stage following the overwhelming response to his MIDAS Seminar Series appearance preceding the 2020 election. David is the Head of Data Science at OpenLabs, a non-profit research lab using data science to provide products for progressive organizations. He cofounded Civis and worked as its Director of Political Data Science. He also worked on the Obama campaign to develop their election forecasting engine the “Golden Report”.
David will talk about what Data Science has to say about the direction American politics is going and how it is being used in US campaigns.
Research Talks: Tuesday, November 15
Matthew D. Shapiro, Survey Research Center and Economics Department, University of Michigan
We demonstrate a machine learning (ML) procedure to estimate hedonic price indices at scale from item-level transaction and product characteristics data. Our procedure incorporates state-of-the-art approaches from hedonic econometrics into a ML framework. Applying our methodology to the Nielsen Retail Scanner data set, we estimate a large hedonic adjustment to the Tornqvist index for food product groups, which reduces cumulative inflation over the period 2006q4–2015q4 by more than half. These results suggest that quality improvement via product turnover is important even in product groups that are not normally considered to feature rapid technological progress.
Xuan Lu, School of Information, University of Michigan
Wei Ai, College of Information Studies, University of Maryland
Zhenpeng Chen, Peking University
Yanbin Cao, Peking University
Qiaozhu Mei, School of Information, University of Michigan
Emotions at work have long been identified as critical signals of work motivations, status, and attitudes, and as predictors of various work-related outcomes. When more and more employees work remotely, these emotional and mental health signals of workers become harder to observe through daily, face-to-face communications.
The use of online platforms to communicate and collaborate at work provides an alternative channel to monitor the emotions of workers. This paper studies how emojis, as non-verbal cues in online communications, can be used for such purposes. In particular, we study how the developers on GitHub use emojis in their work-related activities. We show that developers have diverse patterns of emoji usage, which highly correlate to their working status including activity levels, types of work, types of communications, time management, and other behavioral patterns. Developers who use emojis in their posts are significantly less likely to dropout from the online work platform. Surprisingly, solely using emoji usage as features, standard machine learning models can predict future dropouts of developers at a satisfactory accuracy.
Understanding the mechanism of the correlations and the predictive power of emojis requires a comprehensive understanding of emoji usage in multiple remote work contexts, which calls for theories and methodologies from disciplines such as organizational behavior and psychology. This work can also be generalized to studies of mental health issues in remote work and online education. What are the purposes of using emojis in different scenarios? What kinds of effects do emojis make in work-related communications? What’s the relation between emoji usage and workers’ mental status, and how to verify it? More generally, what kind of research questions can emojis help to answer in different research domains? Cross-disciplinary collaborations would help address such questions.
Winston Wu, Department of Electrical Engineering and Computer Science, University of Michigan
Lu Wang, Department of Electrical Engineering and Computer Science, University of Michigan
Rada Mihalcea, Department of Electrical Engineering and Computer Science, University of Michigan
Fairy tales are one of the most important cultural and social influences on children’s lives. Stereotypes contained in these fairy tales have the potential to influence the rest of our lives. The study of biases in children’s stories and fairy tales has largely been limited to a handful of languages around the world. In this study, we investigate over 850 fairy tales across 22 different cultures, identifying and characterizing differences in stereotypes, such as gender bias and agency in events, that may have been instilled at a young age.
Kevyn Collins-Thompson, School of Information, University of Michigan
Yulia Sevryugina, University of Michigan Library
Our project’s overall research aim is to explore effective methods for addressing readability difficulties that science, technology, engineering, and math (STEM) field students experience when reading scholarly sources. Toward that goal, we are investigating pedagogical practices and resources for effective STEM reading together with novel machine learning-based technologies that support better reading comprehension and retention. Example of the latter include personalized measures of reading difficulty for advanced STEM content, deep learning approaches for finding text passages that are most helpful for learning the meaning of a target concept, and eye-tracking-based predictors that can analyze word-level reading patterns across a document for gaining insight into the ease or difficulty of a text passage.
The field we specifically focus on is biochemistry, the branch of science that explores the chemical processes within and related to living organisms. It brings together biology and chemistry and is at the core of many engineering solutions, not to mention its particular importance during the current coronavirus pandemic. We are actively working with undergraduate and graduate level courses in the Department of Chemistry, where we gather new datasets from classroom reading assignments and user interaction studies in order to comprehend the complex taxonomy of biochemical knowledge, and how to understand and support student engagement with scientific literature. Our presentation will summarize our recent work in progress and early results.
Each year, MIDAS funds a number of innovative and high-impact data science and AI research projects. The project teams will give the audience an overview of their work.
PODS Showcase
The MIDAS Propelling Original Data Science (PODS) grant strongly encourages works that transform research domains through data science and AI, works that improve the reproducibility of research, and works that promise major impact and potential for significant expansion.
Nikola Banovic (Computer Science and Engineering)
Using bibliographic data, studies have reported that female scholars tend to produce fewer papers and attract fewer citations than male scholars, indicating that women in science underperform in terms of scholarly productivity and impact. I argue that such findings are likely based on flawed data in which female authors are not properly identified. Specifically, female scholars may have changed their last names after marriage and have used the changed names in publications instead of their maiden names used in publications authored before marriage. As none of existing bibliographic data services consolidates author entities with different last names, entities of female authors who change names are inevitably split into different entities – one with a maiden name and the other with a marital name. This means that publications and citations of female authors who have used different names are likely undercounted, possibly leading to under-evaluation of their scholarly productivity and impact. This issue can hinder fair evaluation of women in science as female researchers are increasing in number while small fraction of women is found to retain their maiden names. To address the issue, this project will develop a machine learning method to consolidate female author entities in bibliographic data, thus promoting fair evaluation of women in science (> Responsible Research Pillar). Under the PODS grant, first, PI will create large-scale labeled data to train algorithmic models to merge the same female author entities split under different names (> Data Pillar). Then, PI will implement the models on author entities recorded in PubMed which indexes research papers in biomedicine (> Data Pillar), and demonstrate how the correct identification of name-changed female authors can lead us to different understanding of research productivity and citation-based impact of female scholars in the field where almost half of scientists are estimated to be female (> Analytics Pillar). Based on this case study and the algorithmic method, PI will apply for grants from funders such as the NSF to expand the PODS project into a large-scale, cross-field project (> Follow-on Expansion). The findings derived from this project will enable science community and policy makers to correctly characterize the research productivity and impact of female scholars and to implement effective supports and policies to promote fairness and equity for women in science (> Future Impact). A tool that implements the newly developed method will be shared under the UM license for reuse, validation, and improvement with AI researchers (> Contribution to UM data science and AI research ecosystem)
Stephen Smith, Associate Chair, Department of Ecology and Evolutionary Biology, Professor of Ecology and Evolutionary Biology and Associate Curator, Ecology and Evolutionary Biology, College of Literature, Science, and the Arts
William Weaver, College of Literature, Science, and the Arts
While genomic data has revolutionized the biological sciences, data that record physical attributes of organisms remain limited due to the challenges of morphological data gathering techniques. Herbaria contain a staggering wealth of historical biodiversity in the form of specimens and their associated information. However, lack of access constrains the type and scale of research that can leverage these specimens. Recently, most herbaria have undertaken the immense task of digitizing their collections, allowing for images of the specimens to be searchable and easily accessible. However, collecting trait, morphometric, and phenotypic data from digitized specimens remains laborious and limiting. Large-scale genomic analyses are increasingly common, but large-scale morphometric studies are rare due to the time-intensive nature of data collection. We have demonstrated with our software package ‘LeafMachine’ that recent advances in machine learning models and computer vision methods are capable of rapidly extracting useful data from digitized specimens. Nevertheless, major challenges remain. The proposed project will create a deployable open-sourced software package enabling researchers to efficiently process herbarium specimen images. This plays a crucial role in the development of the “virtual herbarium” where the goal is to have rich data accompany each specimen, extending the usefulness of collections in large-scale research projects. We will also leverage the world class herbarium maintained by the University of Michigan to test and validate our software at scale while also significantly increasing the research impact of its specimens, in line with the emerging pillar. This connection to the UM herbarium will strengthen relationships of diverse data-science resources and capabilities on the UM campus. The developments made here also will facilitate future expansion beyond herbarium images to those collected by citizen scientists stored in public databases like iNaturalist and even twitter and instagram. This proposal directly addresses both the Emerging pillar and Methodological foundations pillars
Veronica Perez-Rosas, Assistant Research Scientist, Electrical Engineering and Computer Science, College of Engineering
Kenneth Resnicow, Irwin M Rosenstock Collegiate Professor of Public Health, Professor of Health Behavior and Health Education, School of Public Health and Professor of Pediatrics, Medical School
Rada Mihalcea, Janice M Jenkins Collegiate Professor of Computer Science and Engineering and Professor of Electrical Engineering and Computer Science, College of Engineering
In 2019, 24% of American adults with mental health issues reported unmet treatment needs. Among several other reasons, this can be largely attributed to the current shortage of mental health workers. The situation is also exacerbated by recent issues such as the COVID-19 pandemic and mental health providers burnout. While there is an increasing need for mental health treatment there are also important barriers to the rapid and e↵ective training of mental health practitioners such as the need of extensive clinical supervision and the laborious process this entails. AI technology holds the promise to address such challenges by providing low resource and cost e↵ective opportunities for training counselors to practice and receive real-time evaluative feedback. Current strategies for counselor training rely on monitoring and recording live video interactions, which are then manually evaluated to provide constructive feedback. However, this feedback is usually not immediate as it requires an expert instructor to watch and evaluate each recording. In this project, we seek to build languagebased evaluative tools to provide timely feedback to counselors in training while they learn to formulate responses to clients’ statements. We will focus on reflective listening, i.e., the ability to understand and reflect on what the patient is saying. We plan to use Natural Language Processing (NLP), to build a system able to (1) measure the quality of a reflection formulated by a counseling student in response to a patient statement by providing a reflection accuracy score; and (2) suggest rewritings when responses do not adhere to proper counseling style. Our project aligns with the MIDAS analytics pillar, as we will use AI methods to build language tools to enhance current learning strategies used in the training of future counselor professionals, which in turn will have a positive impact on the current surge of mental health services.
Jie Liu, Assistant Professor of Computational Medicine and Bioinformatics, Medical School and Assistant Professor of Electrical Engineering and Computer Science, College of Engineering
Our knowledge regarding the human genome has been exponentially increasing, driven by the ever-evolving biotechnologies that characterize the human genome from different perspectives. A major source of our knowledge about the human genome comes from direct measurements and annotations of different genomic elements, exemplified by a number of ground-breaking consortia including the ENCODE project, the Roadmap Epigenomics project, the GTEx project, the 4D Nucleome project, and the HuBMAP project. While each of these consortia has a dedicated Data Coordinate Center and a data portal, these consortium datasets are usually tabular-structured, heterogeneous, and sparse, and as a result, the knowledge accumulated from individual consortia is isolated. Another source of our knowledge regarding the human genome comes from individual research labs, which is usually hypothesis-driven and captured in the biological literature. However, the ever-growing biological literature is being stored as unstructured text, and we do not have an infrastructure to extract knowledge buried in the literature. Consolidating two knowledge sources is even more challenging. To tackle these challenges, we aim to develop an open knowledge network for navigating and embedding our ever-growing knowledge regarding the human genome. We will adopt domain knowledge and use cutting-edge machine learning approaches to improve entity and relation extraction from genomic literature, and consolidate with our existing GenomicKB knowledge graph. We will also improve genomic literature search and navigation in the light of our knowledge network.
Margaret Reuter, Research Fellow, Biomedical Engineering, College of Engineering and Medical School
Rudy Richardson, Dow Professor Emeritus of Toxicology, Professor Emeritus of Environmental Health Sciences, School of Public Health and Associate Professor Emeritus of Neurology, Medical School
Sriram Chandrasekaran, Assistant Professor of Biomedical Engineering, Medical School
Pathogens are becoming progressively drug resistant, yet drug discovery methods have failed to produce new classes of antimicrobials for decades. As increasingly pathogenic strains of diseases emerge, there is an urgent need to identify effective therapies from existing U.S. Food and Drug Administration approved drugs. Multi-drug regimens are already being used to fight antibiotic resistance, but they are often chosen empirically, leading to suboptimal treatment outcomes, and spread of resistance. Using a unique combination of structural molecular docking, chemogenomic studies, and machine learning algorithms, we will create a tool for developing effective drug combination therapies and investigate the biochemical principles that govern drug interactions and mechanisms of action. Due to the flexible, multiscale, and hybrid nature of our model, we will be able to examine many combinations, infeasible to interrogate via physical experiments due to cost and time. Secondly, the model will enable us to explore more deeply the underlying biological and chemical factors that influence synergy for better design of drug combination therapy.
Negar Farzaneh, Research Investigator, Emergency Medicine, Medical School
Hamid Ghanbari, Assistant Professor of Internal Medicine, Medical School
Kevin Ward, Medical School
Sardar Ansari, Research Assistant Professor, Emergency Medicine, Medical School
The objective of this project is to develop a multi-label classifier that captures the dependency between different output labels as well as the uncertainty about the ground truth labels in the context of electrocardiogram (ECG) classification. ECG is the primary test for cardiovascular diagnosis, and while automated ECG analysis models are used clinically, they have several flaws, often resulting in inaccurate output. First, the models do not account for hierarchical dependencies among cardiac diseases. For example, both “ectopic atrial tachycardia” and “multifocal atrial tachycardia” share the same “atrial tachycardia” parent disease, but this relationship is not accounted for when using a conventional multi-label classifier, which assumes all classes are equally distinct and independent. Second, the ground truth labels from diagnostic statements often reflect clinician doubts, but current deep learning models ignore these doubts and treat uncertain labels as being a definitive “presence” or “absence” of a disorder. Consequently, we propose to overcome these obstacles by developing novel datadriven diagnosis models, leveraging a unique cohort of >2.15 million ECGs collected at Michigan Medicine. Specifically, we will develop a novel deep learning classifier that takes the hierarchical relationships into account. We will also use a soft (vs. hard) labeling approach to leverage information regarding uncertainty in the model. This research is aligned with the MIDAS “Analytics” and “Emerging” pillars by developing a decision analytic that can precisely determine multiple cardiac diseases, which will improve cardiovascular disease diagnosis and prevent clinical mismanagement resulting from ECG model inaccuracies. This PODS award will lay the necessary groundwork for us to submit a future NIH grant proposal to develop a comprehensive, fully automated cardiac decision support system. Moreover, we will disseminate our findings to other researchers at UM and beyond via presentations and publications.
Joyce Penner, Ralph J Cicerone Distinguished University Professor of Atmospheric Science and Professor of Climate and Space Sciences and Engineering, College of Engineering
Xianglei Huang, Professor of Climate and Space Sciences and Engineering, College of Engineering
Yang Chen, Assistant Professor of Statistics, College of Literature, Science, and the Arts
The largest uncertainties in climate forcing are associated with the forcing by atmospheric aerosols. Uncertainties in aerosol climate forcing are estimated from the spread in forcing estimates in global models and/or observations. The uncertainties in these estimates have remained large primarily because there are no direct estimates of forcing based solely on observations and the many model processes required to estimate forcing are treated differently in different models. As a result, a given model may fit some types of data, while other models fit other data. So far, it has been impossible to understand the causes of the differences in models and thereby to decrease the spread in forcing estimates. This project seeks to develop a datadriven method that will help ascertain why models differ, and ultimately, what may be needed to correct models and deliver estimates of the climate forcing by aerosols that agree more widely. In this work we propose to build a feedforward neural-network emulator that uses inputs from the aerosol/climate model from Penner’s group that are adjusted to better fit the available observations. This will allow us to determine which aspects of the aerosol/climate model need to be improved. We will also work with collaborators at ETHZ to build a similar emulator and compare which aspects of their model need to be adjusted to better fit the observations. The hope is that this will allow the improvement of processes in the two models, and, consequently, allow the two model predictions of climate forcing to converge. A follow-on project will enlist other aerosol modeling groups. This will ultimately allow improvements to all models and thus lead to more cohesive estimates of climate forcing, thereby reducing its uncertainty. The proposed study fits the Responsible Research Pillar and the Emerging Pillar for the 2022 PODS grant solicitation.
Amie Gordon, Assistant Professor of Psychology, College of Literature, Science, and the Arts and Faculty Associate, Research Center for Group Dynamics, Institute for Social Research
Elizabeth Eve Bruch, Associate Professor of Sociology, Associate Professor of Complex Systems, College of Literature, Science, and the Arts and Research Associate Professor, Population Studies Center, Institute for Social Research
Supportive relationships are one of the most robust predictors of well-being and longevity and, thus, a key area for research and intervention. However, we know little about the processes through which people enter into committed relationships and how partner choices are associated with the challenges people encounter in maintaining their relationships over time. To address this gap in knowledge, we need longitudinal data from large groups of people as they enter and then sustain or dissolve relationships. Dating apps have detailed information on how people enter into relationships; however, researchers do not typically have access to the data collected by these apps. In addition, existing dating apps only track relationship formation, not relationship maintenance. Therefore, we propose creating a research-based dating app that will track individuals’ dating decisions and relationship behaviors over time. By launching this data collection tool in the University of Michigan (U-M) population, we will create a valuable resource that can help answer questions regarding preferences and choice, partner selection, relationship formation, and relationship maintenance. This project aligns most closely with the Data Pillar, as it will result in a novel and important data source for understanding human behavior. We also anticipate working with computer science collaborators to develop a privacy-preserving synthetic dataset as a good model of how to do open-source, transparent, privacy preserving work using detailed observational data from Apps, which aligns with both the Data Pillar and the Responsible Research Pillar. In addition, this project aligns with the Analytics Pillar because it provides opportunities to use cutting-edge methods in choice modeling and natural language processing to better predict human behavior and important health-related outcomes (e.g., relationship status, relationship quality). This project has potential for broad impact and the improvement of society, as insights about relationships and data privacy can benefit all populations.
Arun Agrawal, Samuel Trask Dana Professor, Professor of Environment and Sustainability, School for Environment and Sustainability, Faculty Associate, Center for Political Studies, Institute for Social Research, Professor of Political Science, College of Literature, Science, and the Arts and Professor of Public Policy, Gerald R Ford School of Public Policy
Ines Ibanez, Professor of Environment and Sustainability, School for Environment and Sustainability, Professor of Ecology and Evolutionary Biology, College of Literature, Science, and the Arts, Professor of Environment, Program in the Environment, School for Environment and Sustainability and College of Literature, Science, and the Arts and Adjunct Professor of Biological Station, College of Literature, Science, and the Arts
Yang Chen, Assistant Professor of Statistics, College of Literature, Science, and the Arts
Changing human behavior has substantial potential to slow and even alter degradation trends. However, little is known about the potential and the effectiveness of changing human behavior over sustainability outcomes. Mandatory lockdowns and calls for voluntary restraint were key interventions to reduce disease exposure risks in the early stage of COVID-19. The resulting global reductions in human mobility constituted an unprecedented and massive natural experiment in how changes in human behaviors affected sustainability. We have analyzed the effects of mobility restrictions on forest fires across the Amazon basin using large scale remote sensing datasets on mobility and fires. We found that mobility restrictions initially led to a decline in fires, but fires rebounded in Amazon forests within 30 days to levels exceeding preCOVID-19 lockdown levels. The resulting research is now under review at a prominent journal. We seek support from MIDAS to expand our current work on a global scale. Spatially, we will examine how mobility restrictions affect the incidence of forest fires in other regions and forest types, and if patterns in the Amazon are generalizable globally. Thematically, we will analyze effects of mobility restrictions on air pollution in the immediate to longer term and across rural to urban gradients. Bayesian mixed effects models with spatiotemporal autocorrelations in conjunction with large-scale datasets will help generate a deeper understanding of the durability of sustainability outcomes associated with human behavioral changes. The proposed study aligns with all five research pillars of MIDAS, but particularly so with the Data, Analytics, and Emerging pillars. We will be able to differentiate the effects of COVID-19 lockdowns on forest fires and air pollution across sectors; develop a full proposal for NSF’s DISES program on persistent effects of human behavioral changes; contribute to UM’s research ecosystem by generating usable, publicly available COVID-Fires and COVID-Air Pollution datasets.
Qing Qu, Assistant Professor of Electrical Engineering and Computer Science, College of Engineering
Pei-Cheng Ku, Associate Chair, Department of Electrical and Computer Engineering and Professor of Electrical Engineering and Computer Science, College of Engineering
Spectroscopy is one of the most important and widely utilized techniques in science and technology, with broad applications in chemistry, life science, microbiology, food industry, biomedical sensing (lab-on-a-chip), environmental monitoring, pharmaceutical research, cosmetic industry, and quality control. For example, fluorescence spectroscopy is a crucial resource for viral detection and vaccine research, two needs of great societal importance during the pandemic. This research aims to develop machine learning methods for co-designing an on-chip spectrometer that can enable a highly miniaturized and portable sensing platform for UV-VIS, fluorescence, and chemi-/electro-luminescence spectroscopy application. The major challenges lie in the spectrum reconstruction with a limited number of encoders/photodetectors, which result in challenging machine learning problems and the existing method performs poorly. The collaboration between Qu (with expertise in machine learning) and Ku (with expertise in semi-conductor devices) will resolve the challenge by developing new machine learning methods, which learn more precise models of the data acquisition process and provide more efficient reconstruction algorithms, leading to faster and more accurate spectrum recovery. In return, the developed learning methods will provide guidance for the better design of the sensing platform.
posters with students as first authors are entered automatically in the poster competition for cash awards
Dr. H. V. Jagadish, Edgar F Codd Distinguished University Professor and Bernard A Galler Collegiate Professor of Computer Science and Engineering; MIDAS Director
All attendees invited.
Keynote Speakers
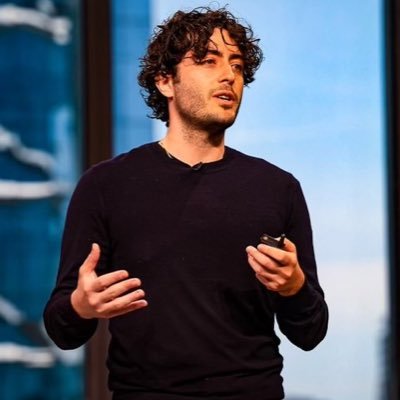
David Shor
Head of Data Science
OpenLabs R&D
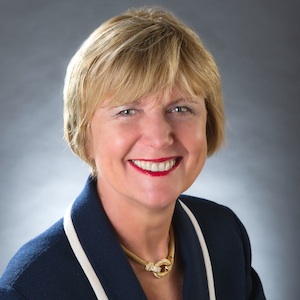
Suzanne R. Bakken
Professor of Biomedical Informatics and Alumni Professor of the School of Nursing, Columbia University
Editor-in-Chief, Journal of the American Medical Informatics Association
Selected Organizations Represented at the 2022 Summit
- Advanced Research Computing
- Aerospace Engineering
- AI Lab
- Anesthesiology
- Anthropology
- Applied and Interdisciplinary Mathematics
- Astronomy
- Behavioral Sciences
- Biomedical Engineering
- Biostatistics
- Bold Challenges
- Business
- Chemical Engineering
- Chemistry
- Climate and Space Sciences and Engineering
- Communication and Media
- Computational Discovery and Engineering
- Computational Medicine and Bioinformatics
- Computer Science and Engineering
- Consulting for Statistics, Computing and Analytics Research
- Dentistry
- Digital Studies
- E-Health and Artificial Intelligence
- Ecology and Evolutionary Biology
- Economics
- Electrical Engineering and Computer Science
- Emergency Medicine
- Environment and Sustainability
- Epidemiology
- Ethics, Society, and Computing
- Global REACH
- Government Relations
- Health Management and Policy
- Industrial and Manufacturing Systems Engineering
- Industrial and Operations Engineering
- Information
- Information and Technology Services
- Innovation Partnerships
- Inter-university Consortium for Political and Social Research
- Internal Medicine
- Kinesiology
- Lab Animal Medicine
- Law
- Learning Health Sciences
- Library
- Life Sciences
- Mathematics
- Mechanical Engineering
- Michigan Data Collaborative
- Microbiology and Immunology
- Museum of Art
- Nephrology
- Neurology
- Neuroscience
- Nuclear Engineering and Radiological Sciences
- Nursing
- Obstetrics & Gynecology
- Ophthalmology
- Organizational Studies
- Pathology
- Pediatrics
- Pharmacy
- Physics
- Political Science
- Precision Health
- Psychiatry
- Psychology
- Public Policy
- Radiation Oncology
- Radiology
- Robotics
- Science, Technology and Public Policy
- Social Research
- Sociology
- Software and Data Carpentries
- Statistics
- Survey Research
- Taubman College
- Transportation Research
- Altair
- Amazon Web Services
- Amgen
- Amnesty International
- Ann Arbor SPARK
- Arbor Research Collaborative for Health
- Arkansas Center for Health Improvement
- Brown University
- City of Detroit
- Clemson University
- Detroit Land Bank
- Dickinson College
- Ford Motor Company
- General Dynamics
- General Motors
- Gongos
- Groundspeed Analytics
- Henry Ford Health
- ITHAKA
- Jackson National Life
- JointSpace
- Kettering University
- KLA
- Level X Talent
- Little Caesars Enterprises
- Lucas/McIntosh Communications
- Luna Innovations
- Maxar
- Merit Network
- Michigan State University
- MiddleGround Capital
- Navv Systems
- PathwaysGI
- PPG Industries
- Publicis Groupe
- Rocket Companies
- SalesPage Technologies
- São Paulo State University
- Save the Children International
- State of Michigan
- STATISTICA
- Tempus
- The Brattle Group
- Tinder
- University of Texas at Austin
- US Army Ground Vehicle Systems Center
- Voise
- Wacker Chemical Corporation
- Washtenaw Community College
- WestCap
- William Beaumont Hospital
- Yazaki North America
Program Committee
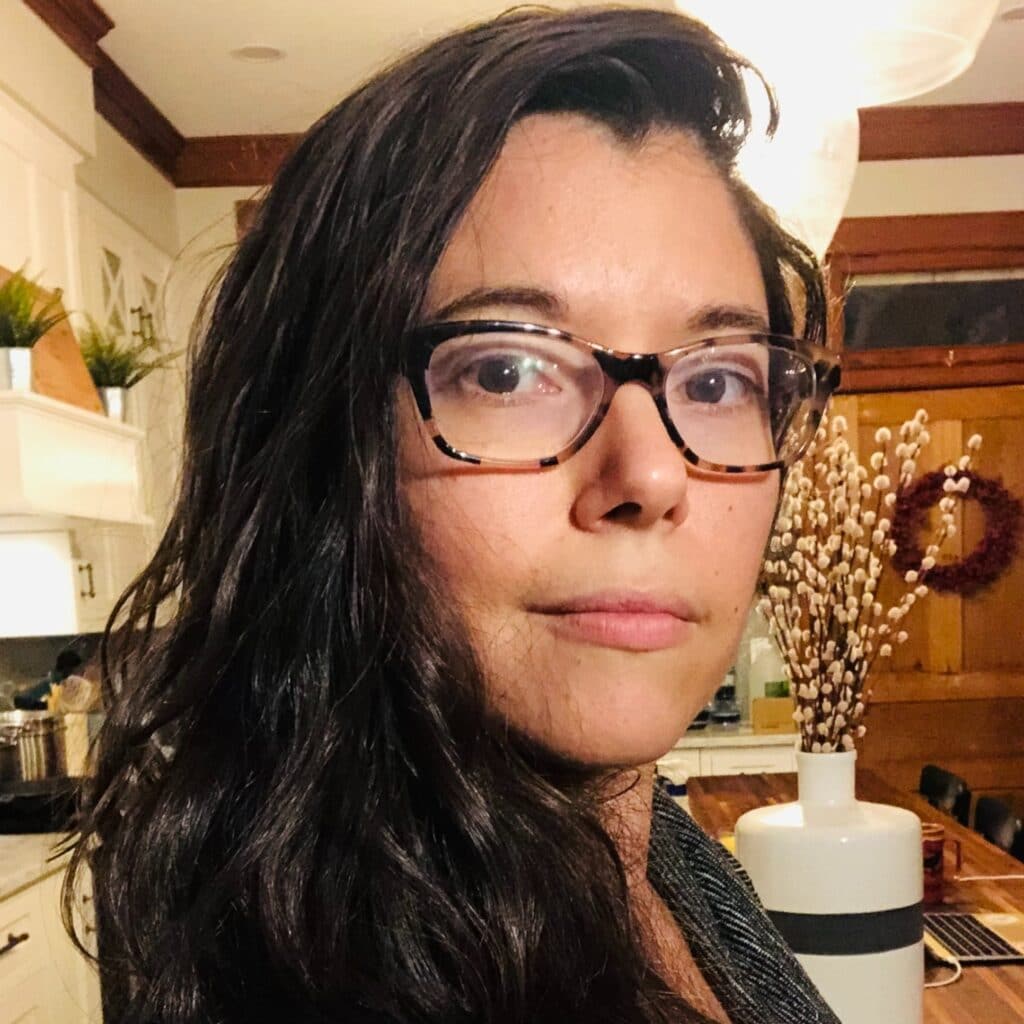
Lia Corrales
Astronomy
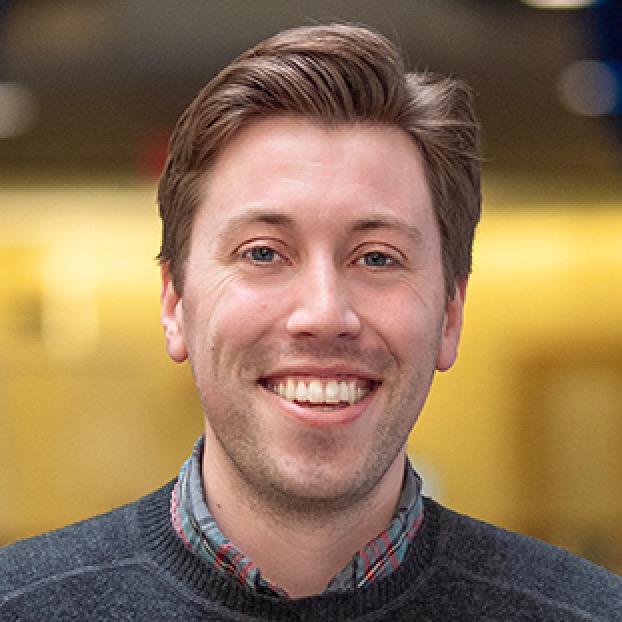
Walter Dempsey
Biostatistics
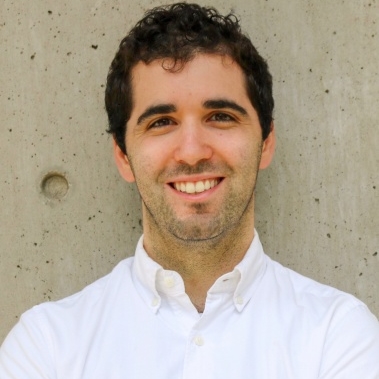
Ben Green
Public Policy
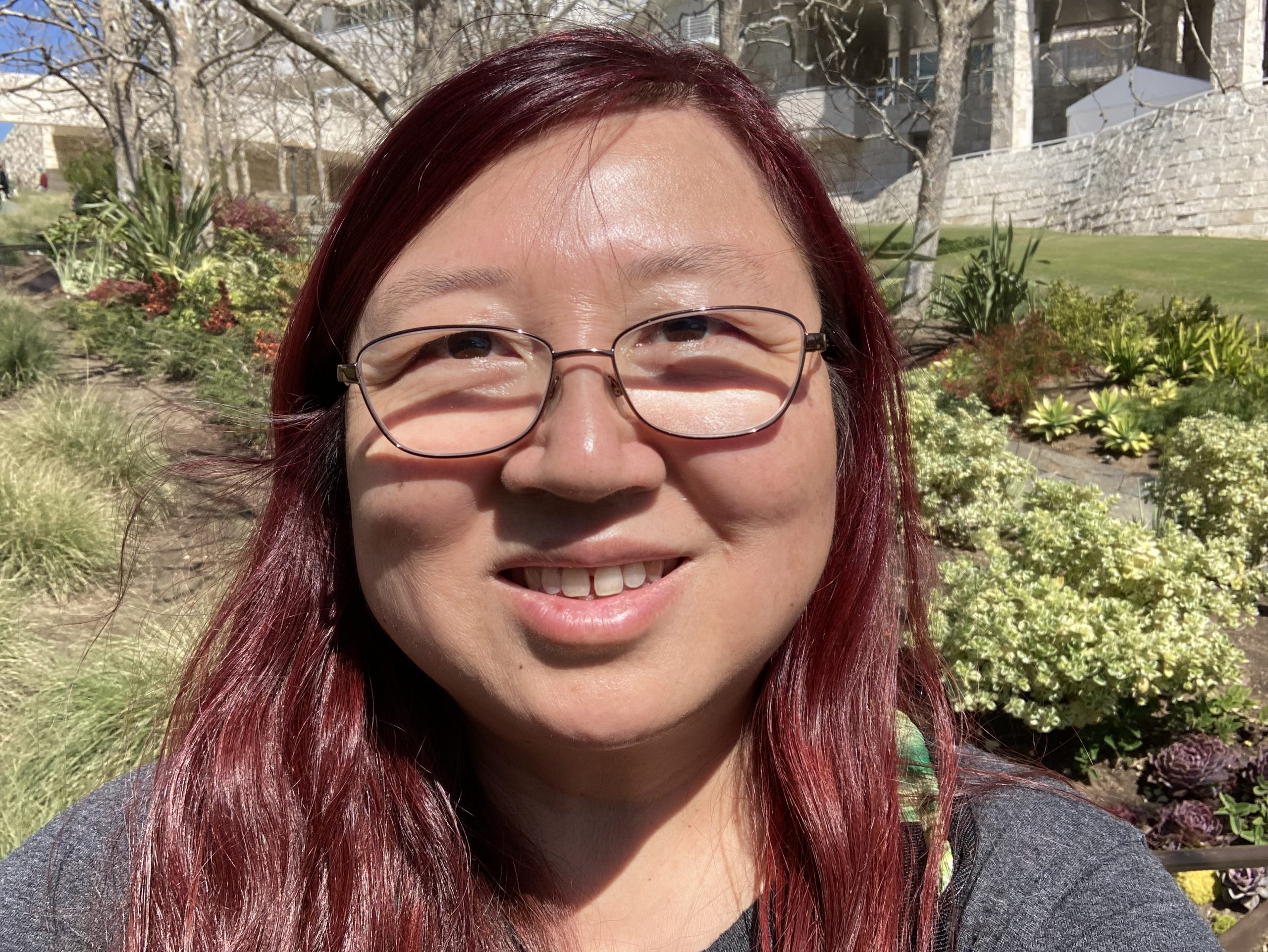
Jing Liu
MIDAS
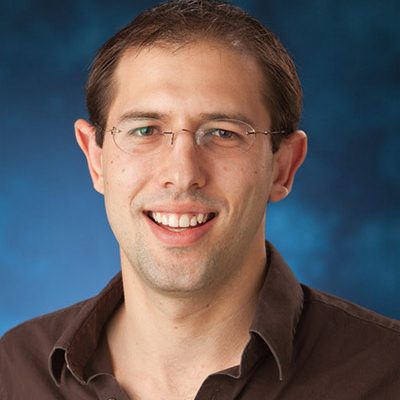
Josh Pasek
Communication and Media
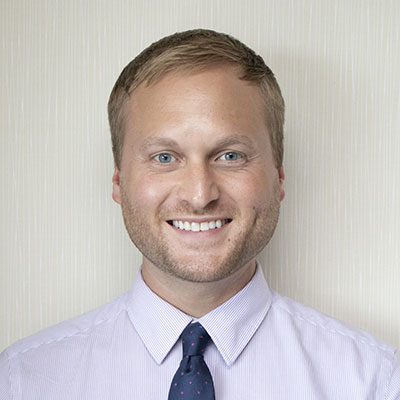
Shane Redman
MIDAS
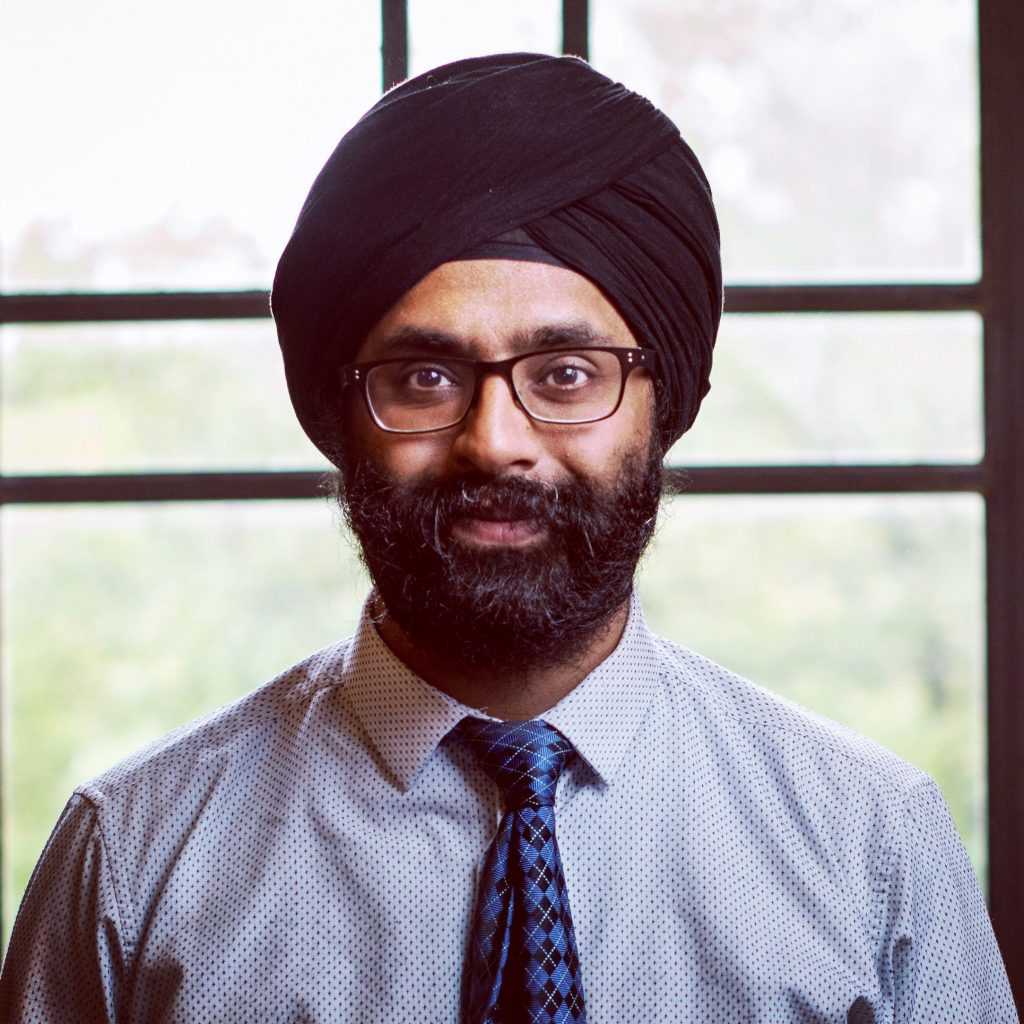
Karandeep Singh
Learning Health Sciences
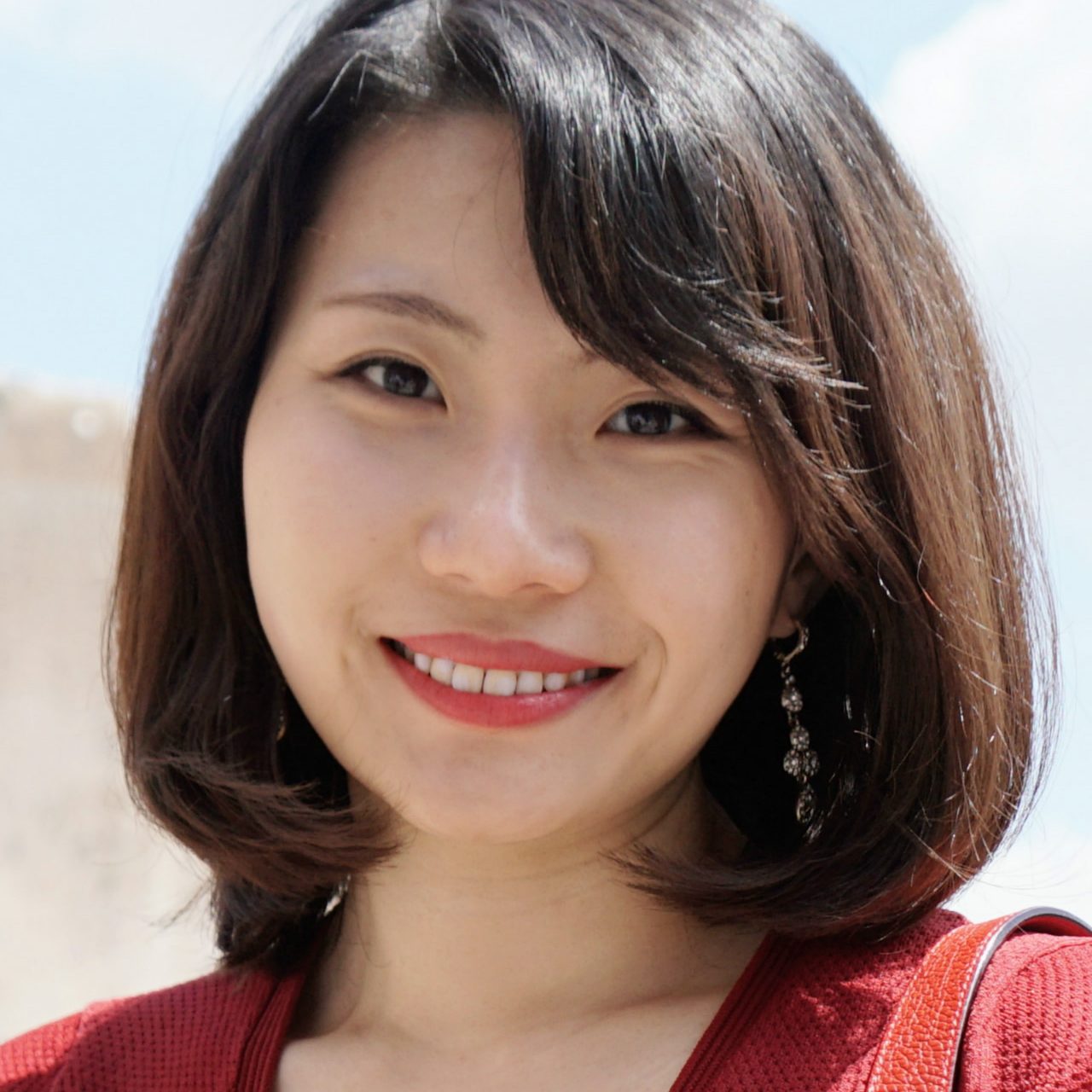
Lu Wang
Computer Science and Engineering
Facility Info
- Rackham Graduate School: 915 E. Washington St., Ann Arbor MI 48109
- Parking – INFO
- There are a limited number of metered parking spaces on Washington Street in front of the building, including an accessible space. There is an accessible entrance in front of the building, as well as in the underground parking garage. The garage is accessed from Huron Street (back of building) and is perfect for active loading and unloading only for vehicles with 90″ or lower clearance. No visitor parking is permitted in the Rackham garage.
- Driving Directions
- All programming will be on the 4th floor, accessible via elevators to the east or west side of the building.
- Accessible men’s restroom (4012M) and women’s restroom (4512W) available on the 4th floor. Accessible, gender-inclusive restrooms available on the 1st floor (1514T) and the 3rd floor (3132T & 3134T).
- Amphitheatre: most of the programming will occur in the Amphitheatre. This room has limited accessible seating via the central, north entrance; additional accessible seating is available and programming will be re-streamed synchronously in the East and West Conference rooms on the same floor. Staff volunteers will be on hand to direct and answer questions.
- No food or drink is allowed in the Amphitheatre.
- Standing room is not allowed in the Amphitheater. Guests will be directed to the East and West Conference rooms if Amphitheater reaches capacity.
- Unfortunately, we are not able to offer a hybrid option at this time for remote participants. Program sessions will be recorded and made available after the Summit. Please allow up to 2 weeks for recordings to be made available. Recordings will include professional transcription and captioning.
Contact Us
If there is an accommodation you would like to discuss with program staff, please send us an email at midas-contact@umich.edu.