Johann Gagnon-Bartsch, PhD, is Assistant Professor of Statistics in the College of Literature, Science, and the Arts at the University of Michigan, Ann Arbor.
Prof. Gagnon-Bartsch’s research currently focuses on the analysis of high-throughput biological data as well as other types of high-dimensional data. More specifically, he is working with collaborators on developing methods that can be used when the data are corrupted by systematic measurement errors of unknown origin, or when the data suffer from the effects of unobserved confounders. For example, gene expression data suffer from both systematic measurement errors of unknown origin (due to uncontrolled variations in laboratory conditions) and the effects of unobserved confounders (such as whether a patient had just eaten before a tissue sample was taken). They are developing methodology that is able to correct for these systematic errors using “negative controls.” Negative controls are variables that (1) are known to have no true association with the biological signal of interest, and (2) are corrupted by the systematic errors, just like the variables that are of interest. The negative controls allow us to learn about the structure of the errors, so that we may then remove the errors from the other variables.
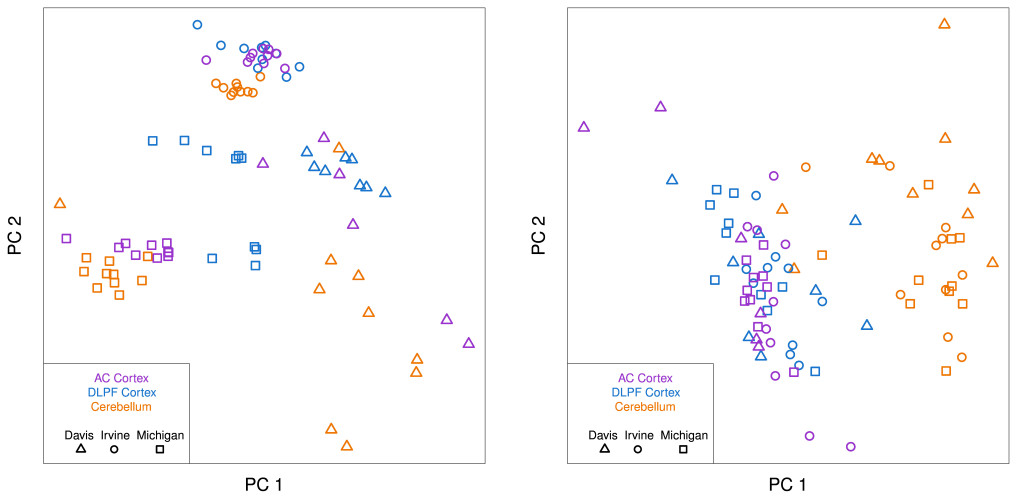