MIDAS announces awardees for the 2024 Propelling Original Data Science (PODS) Grants
The Michigan Institute for Data and AI in Society (MIDAS) announced the awardees of the 2024 round of Propelling Original Data Science (PODS) grants in June. This year, MIDAS collaborated with Microsoft and the Michigan Institute for Healthcare Policy and Innovation to provide more than $700K in funding to fourteen teams across three focused funding tracks for the PODS grants: (1) Data science and AI methodology and application, (2) Accelerating responsible AI research ecosystems, and, (3) AI for Health Policy and Healthcare: Impact & Governance. Read the full press release in the University Record.
The 2024 awardees are:
PODS Track 1: Data science and AI methodology and applications
- WinAI: Propelling UM Soccer with Data-Driven AI
Albert Berahas (College of Engineering) and Raed Al Kontar (College of Engineering) - Human-in-the-loop multi-agent sequential decision-making based Optimal Operation of Power Distribution System
Srijita Das (College of Engineering and Computer Science, U-M Dearborn) and Van Hai Bui (College of Engineering and Computer Science, U-M Dearborn) - Extrapolating with Generative Models for Design of Organic Molecules as Energy Carriers
David Kwabi (College of Engineering), Bryan Goldsmith (College of Engineering), and Yixin Wang (College of Literature, Science and the Arts) - Multimodal Modeling of Cognitive Load at Individual and Team Levels in Acute Care Teams using VR Simulations
Vitaliy Popov (Michigan Medicine), Mohamed Abouelenien (College of Engineering and Computer Science, U-M Dearborn), Michael Cole (Michigan Medicine), and James Cooke (Michigan Medicine) - Combining ecological first principles and AI to better upscale and predict global carbon, nutrient and water cycles on a changing planet
Peter Reich (School for Environment and Sustainability) and Mohammed Ombadi (College of Engineering) - Machine Learning for Automated Fish Detection and Characterization
Katie Skinner (College of Engineering) and Jacob Allgeier (College of Literature, Science and the Arts) - Distributing Expert Attention in Complementary Systems
Sabina Tomkins (School of Information), Derek Van Berkel (School for Environment and Sustainability), Grant Schoenebeck (School of Information), Ariel Hasell (College of Literature, Science and the Arts), and John Ryan (College of Literature, Science and the Arts) - AI-driven Accelerated Optimization for the Design of Sustainable Aviation Fuels
Angela Violi (College of Engineering) - Neural Posterior Estimation (NPE) approaches for fitting high-dimensional stochastic epidemic models to real-world spatiotemporal disease data
Jon Zelner (School of Public Health) and Fan Bu (School of Public Health)
PODS Track 2: Accelerating responsible AI research ecosystems
- A Joint Human-AI Framework for Responsible AI
Rita Chin (College of Literature, Science and the Arts) and H.V. Jagadish (College of Engineering) - Advancing Responsible AI by Rethinking the Roles of Marginalized Communities in the Innovation Lifecycle: Developing the UBEC Approach
Shobita Parthasarathy (Ford School of Public Policy), Ben Green (School of Information) and Molly Kleinman (Ford School of Public Policy) - Innovating, Applying, and Educating on Fairness and Bias Methods for Educational Predictive Models
Christopher Brooks (School of Information), Libby Hemphill (Institute for Social Research and School of Information), and Allyson Flaster (Institute for Social Research) - Evaluating Solutions to the Decline of Online Knowledge Communities
Yan Chen (School of Information) and Qiaozhu Mei (School of Information)
PODS Track 2: Accelerating responsible AI research ecosystems
- Trust, Governance, and Humans in the Loop in Clinical AI
Kayte Spector-Bagdady (Medicine) and Nicholson Price (Law)
Since 2016, MIDAS has been offering funding to U-M faculty, and as of 2023 has already supported 75 teams with more than $12 million in funding to jumpstart their projects, form new collaborations, and secure external funding to expand upon their work. More than 116 projects with $125 million in external funding have resulted from these pilot grants. A list of past MIDAS funded projects can be found here.
For details about this year’s PODS projects, read below:
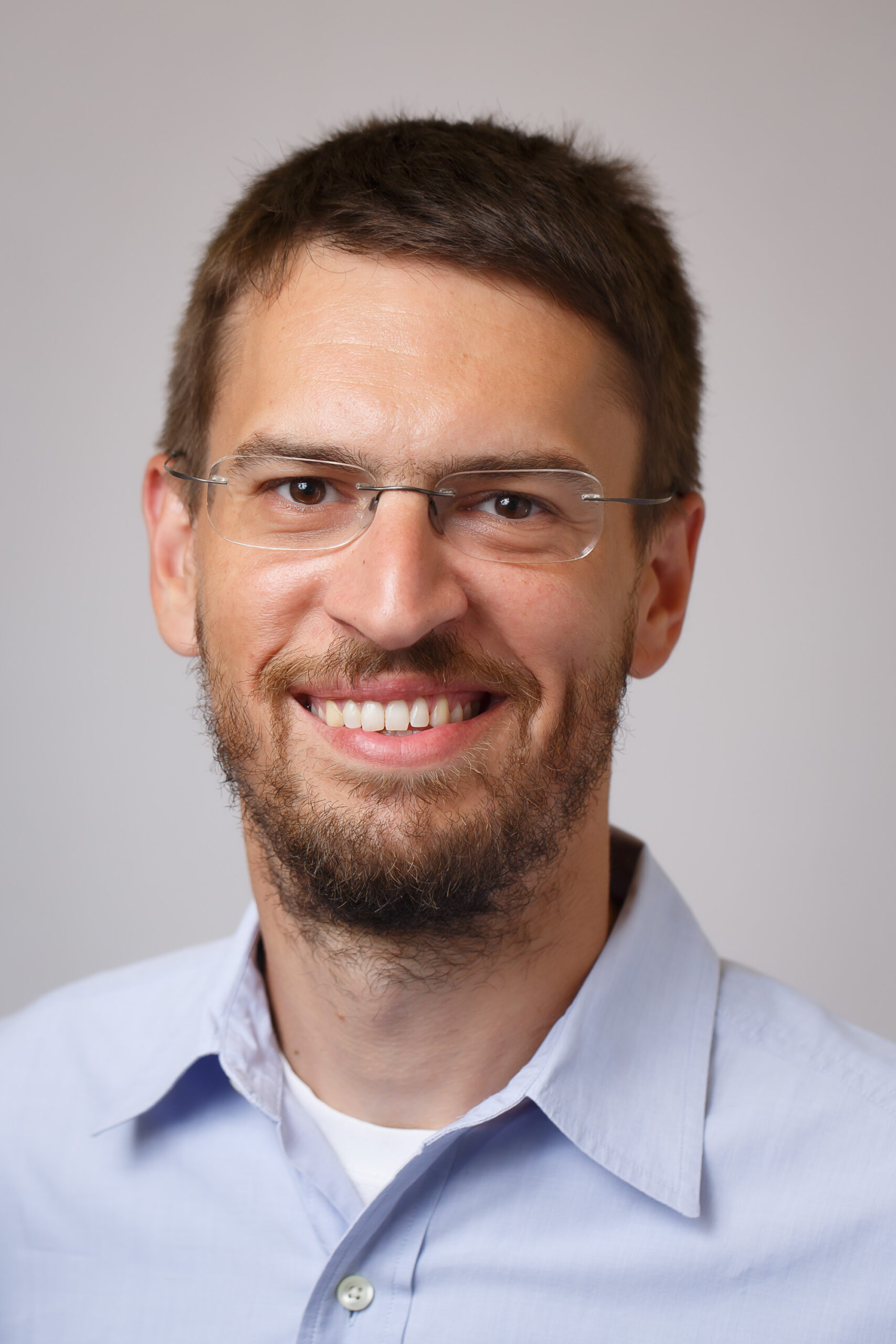
Albert Berahas (College of Engineering) and Raed Al Kontar (College of Engineering):
The WinAI initiative seeks to help the University of Michigan (UM) soccer teams by tapping into the vast amounts of underutilized data in conjunction with cutting edge data science techniques. This proposal outlines a systematic plan to build, pilot and refine next generation innovative models for the UM Women’s and Men’s soccer teams, aiming to optimize tactics, refine team management, prevent injuries, and aid with scouting. WinAI constitutes a progressive, interdisciplinary venture aimed at harnessing untapped data to significantly enhance competitive strategy and player development, ultimately contributing to the UM data science, AI, and athletics ecosystems.
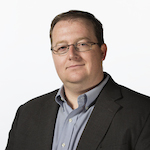
Christopher Brooks (School of Information), Libby Hemphill (Institute for Social Research and School of Information), and Allyson Flaster (Institute for Social Research):
Educational predictive models must be fair in order to ensure that all learners who need support are able to get it. Using 20 years of detailed student-level data from 19 universities and colleges, we demonstrate how institutions can train, share, and reuse predictive models in a way in which learner privacy is protected and the models perform well across learner identity groups. In addition to this large scale study, this project provides open educational materials to further the area of educational data science with the aim of upskilling and enabling educational researchers with state of the art techniques for achieving fair predictive models.
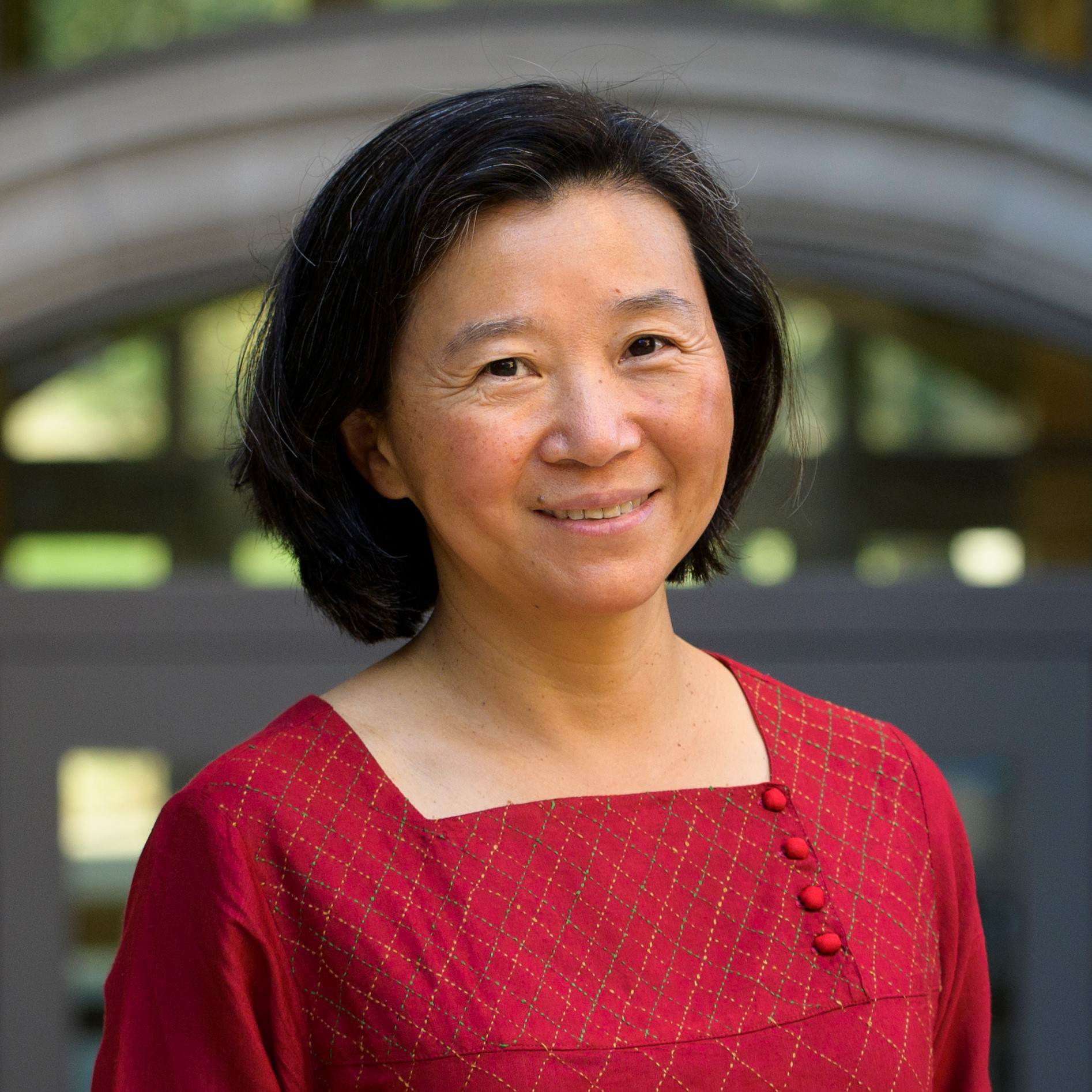
Yan Chen (School of Information) and Qiaozhu Mei (School of Information)
This project explores innovative ways to enhance online knowledge communities like Wikipedia by integrating generative AI tools with human editorial teams. We aim to develop new methods that make it easier for newcomers to join and contribute to these platforms, fostering a more inclusive and dynamic environment. Ultimately, our work seeks to sustain and enhance these essential communities by blending the strengths of both human creativity and artificial intelligence.
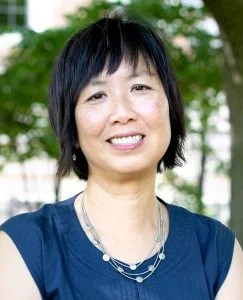
Rita Chin ( College of Literature, Science and the Arts) and H.V. Jagadish (College of Engineering):
Drawing on our cross-disciplinary expertise, our research team will identify the core values and concerns that scholars and developers should consider when building AI systems and engaging in AI research. These will be embodied in a framework of items and scales for responsible conduct of AI research, which will serve as the basis of an AI-specific training course modeled on the University of Michigan’s PEERRS program for responsible conduct of research. We also intend to produce a public-facing Code of AI Ethics akin to the “Code of Medical Ethics” and “Code of Anthropological Ethics.”
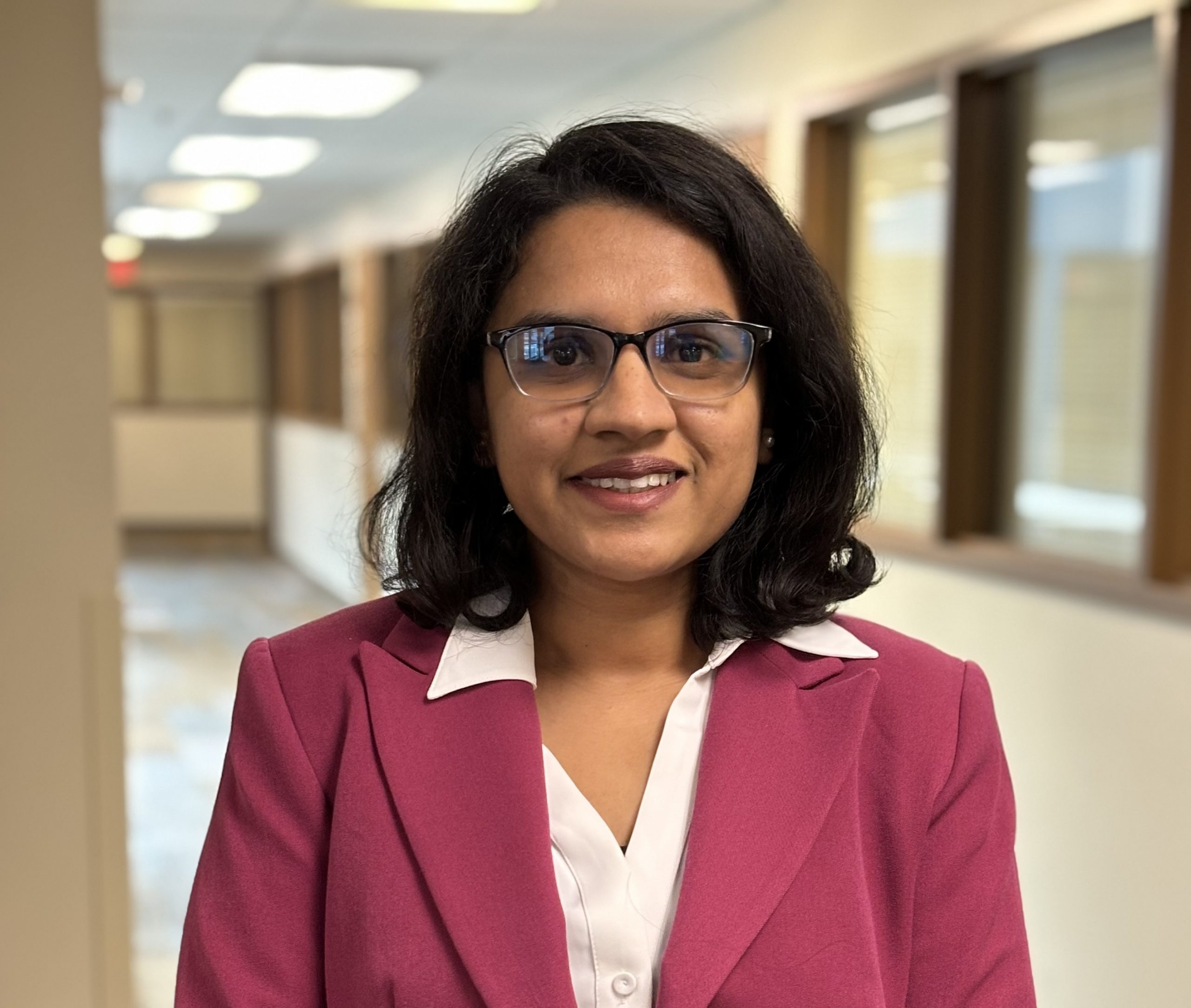
Srijita Das (College of Engineering and Computer Science, U-M Dearborn) and Van Hai Bui (College of Engineering and Computer Science, U-M Dearborn)
The proposal aims at effectively integrating distributed energy resources like batteries, solar panels, etc. into modern power systems using human-in-the-loop sequential decision-making to make these systems safe and reliable while minimizing their environmental impact. We propose to leverage different modalities of advice from humans, which would provide an additional layer of scrutiny and guidance to help these systems learn safe behavior and train faster. Finally, we would also make these systems scalable by developing suitable knowledge transfer mechanisms.
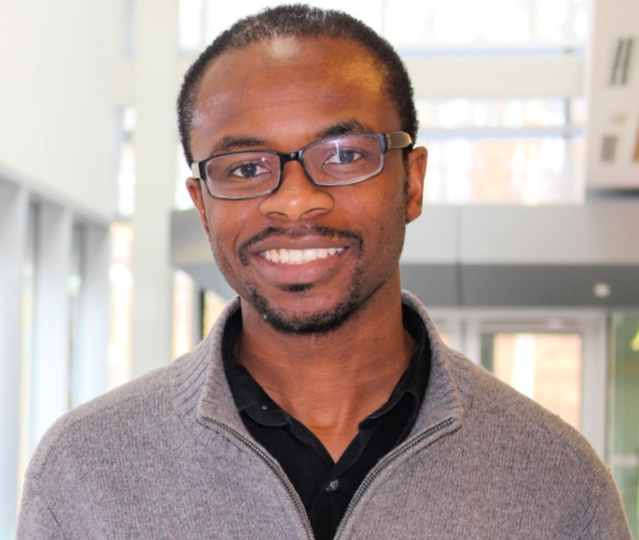
David Kwabi (College of Engineering), Bryan Goldsmith (College of Engineering), and Yixin Wang (College of Literature, Science and the Arts)
The redox-flow battery is a promising new technology for inexpensive storage of electricity on the grid and has the potential to significantly expand our use of renewable (e.g., solar and wind) power. This project will develop and use extrapolative equivariant flow matching (EEFM), a class of models that targets extrapolative generative modeling, for inverse design of organic molecules as energy carriers in these systems.
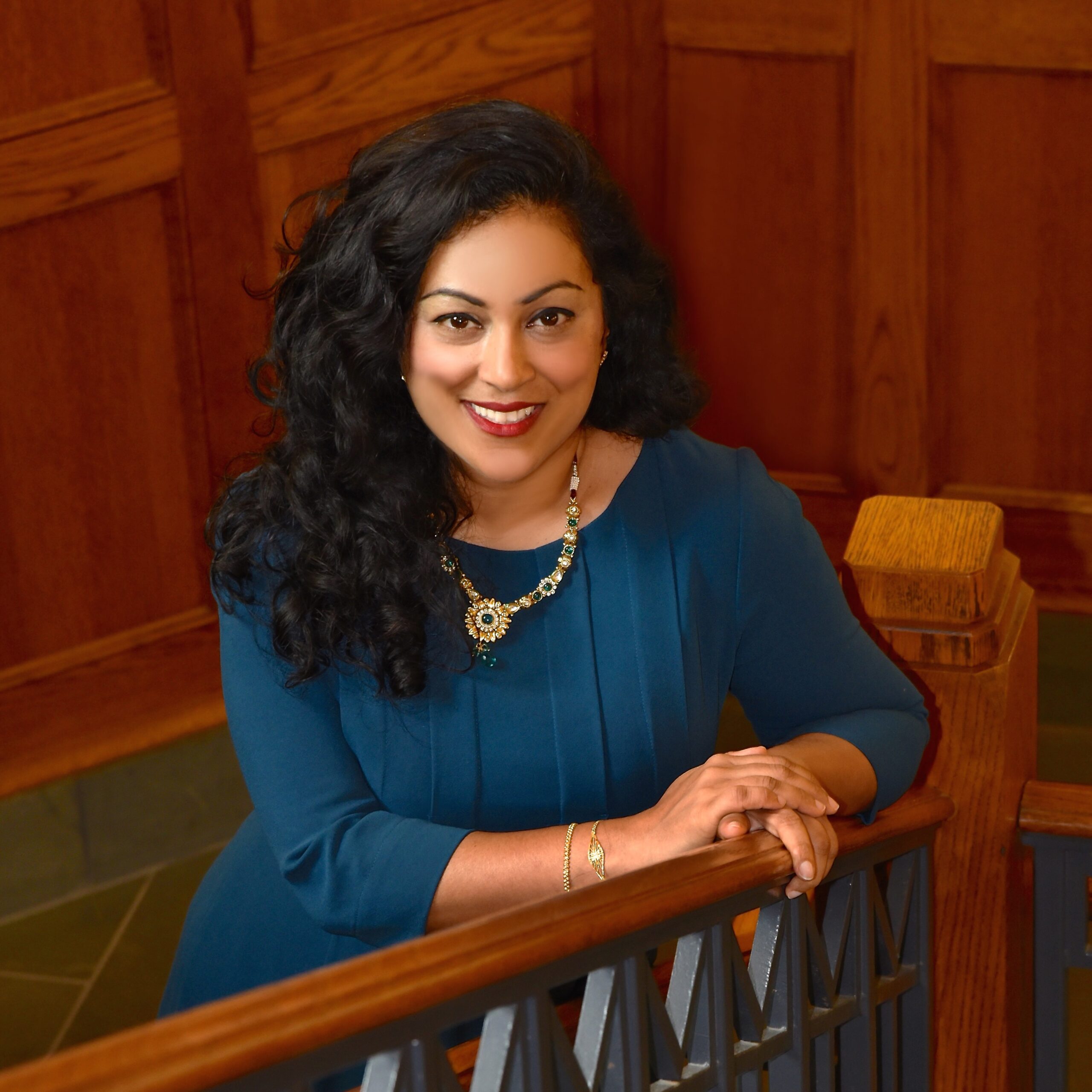
Shobita Parthasarathy (Ford School of Public Policy), Ben Green (School of Information) and Molly Kleinman (Ford School of Public Policy)
This project advances knowledge toward a responsible, and specifically more socially equitable and just, AI research ecosystem by developing and evaluating the novel UBEC approach for the innovation lifecycle, that centers the knowledge and needs of marginalized communities and includes expertise across academic disciplines. Collaborating with local community partners, we produce two kinds of deliverables: 1) technology that is collaboratively designed (e.g., generative AI to help formerly incarcerated people in the greater Detroit metropolitan area understand rules, regulations, and social services relevant to them); and 2) briefs and reports to build civic capacity for participating in AI-related public and policy discourse (e.g., policy briefs on the use of AI in the criminal legal system). This work will also produce best practices for researchers who seek to advance equitable and just AI, at UM and beyond.

Vitaliy Popov (Michigan Medicine), Mohamed Abouelenien (College of Engineering and Computer Science, U-M Dearborn), Michael Cole (Michigan Medicine), and James Cooke (Michigan Medicine)
The emergency department (ED) is a complex environment that is highly susceptible to errors and resultant patient harm because cognitively dense decision-making occurs under information- and time-constrained circumstances. This cutting-edge project addresses this critical issue by combining immersive virtual reality simulations with advanced AI and biosensor technology to detect when acute care teams are approaching cognitive overload during crisis situations. By modeling the factors driving team overload, our research team aims to pioneer new personalized training methods that will enable acute care teams to provide optimal patient care, even in the most extreme circumstances.
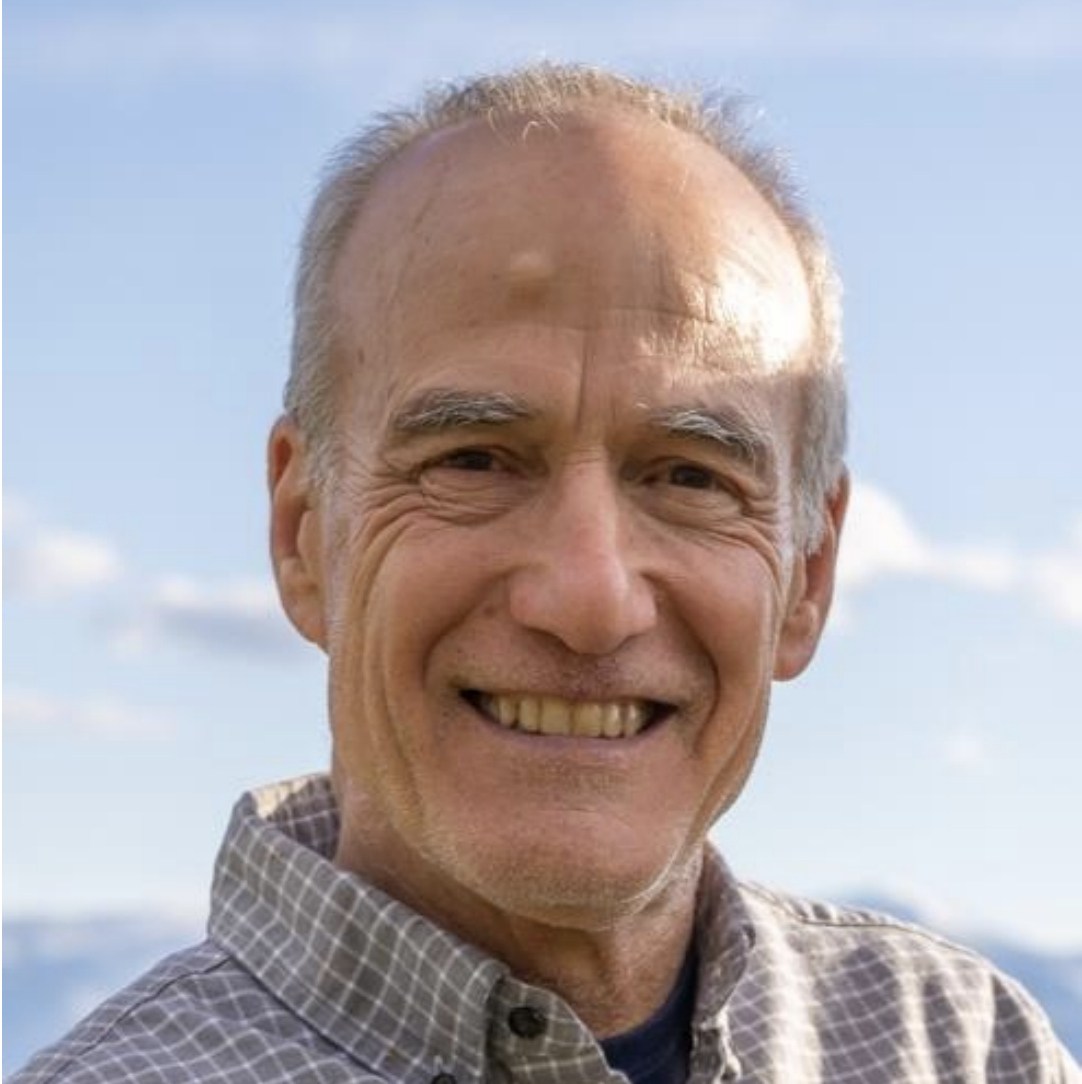
Peter Reich (School for Environment and Sustainability) and Mohammed Ombadi (College of Engineering):
We will use ecological intelligence combined with AI to improve our understanding of the global carbon cycle and our capacity to better model future feedbacks from terrestrial vegetation to the climate system.
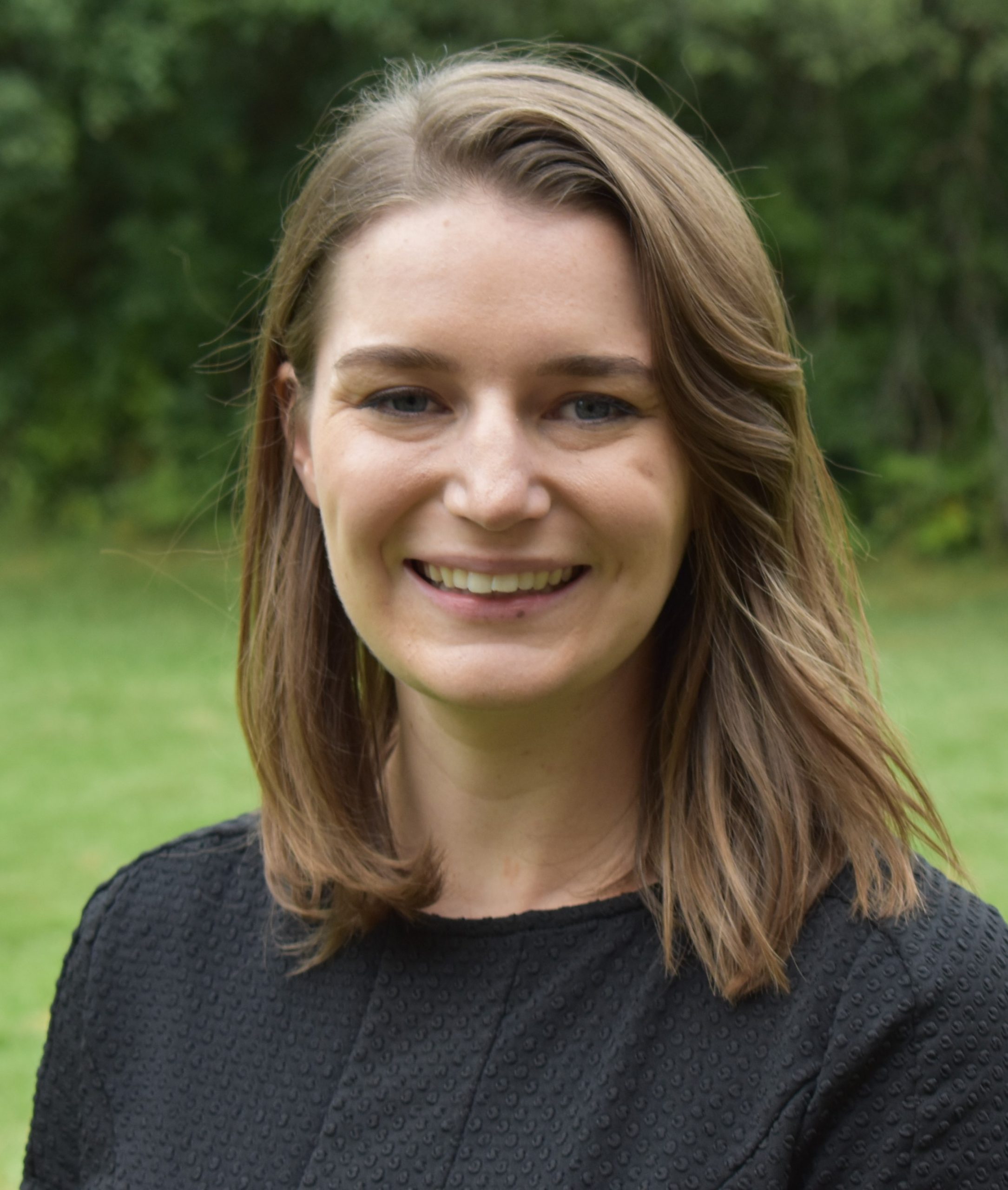
Katie Skinner (College of Engineering) and Jacob Allgeier (College of Literature, Science and the Arts)
This project aims to develop new technology and machine learning methods to enable automated fish detection and characterization. This project will leverage data collected in real environments, including publicly available data, to enable development and evaluation of the proposed system. The future impact of this work will be to advance technology for environmental monitoring efforts in marine environments.
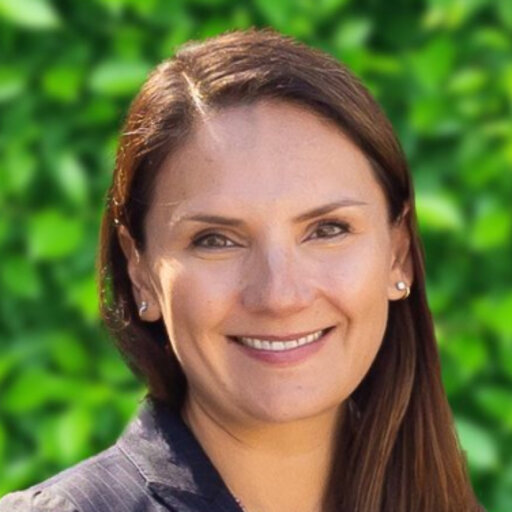
Kayte Spector-Bagdady (Medicine) and Nicholson Price (Law)
A lack of research on the factors and characteristics that make AI trustworthy to patients is a significant problem for ensuring safe and effective AI. Using conjoint and vignette survey experimental designs, in this project we will (1) explore patient preferences and perceived acceptability of trade-offs regarding AI governance, performance, informational disclosure, and clinician oversight; and (2) measure patient preferences and perceived acceptability of trade-offs regarding characteristics of the overseeing clinician relative to AI governance, performance, and informational disclosure. Our overall goal is to inform the creation of trustworthy, patient-centered policies for AI governance.

Sabina Tomkins (School of Information), Derek Van Berkel (School for Environment and Sustainability), Grant Schoenebeck (School of Information), Ariel Hasell (College of Literature, Science and the Arts), and John Ryan (College of Literature, Science and the Arts)
As AI becomes more ubiquitous there are open questions around how to design responsible and complementary human-AI systems. We propose a novel system for Distributing Expert Attention in Complementary Systems. Our system can be used to answer societally urgent questions from digital trace data and will be implemented here to address questions relevant to both biodiversity and climate change.
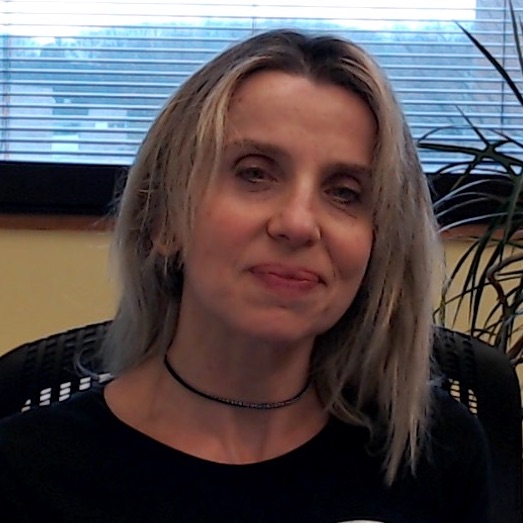
Angela Violi (College of Engineering)
This project seeks to create new designs for Sustainable Aviation Fuels (SAFs) that can be used in current aircraft engines while reducing environmental impact. By utilizing a machine learning framework, we aim to tailor the SAF formulations that uphold the necessary physical characteristics for compatibility with jet engines. Our method stands out by generating and analyzing an extensive range of potential SAF options, ensuring the best versions are both environmentally friendly and perform well, getting us closer to a future of cleaner skies.
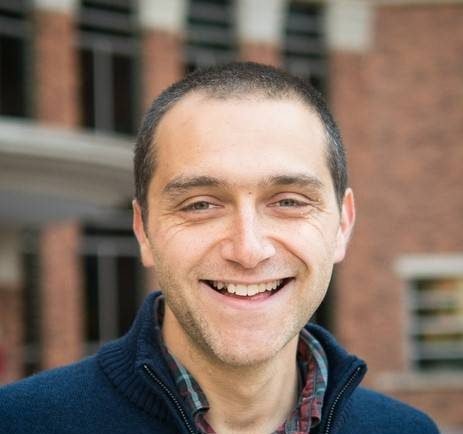
Jon Zelner (School of Public Health) and Fan Bu (School of Public Health)
Stochastic disease models have become a central tool for the practice of epidemiology but calibrating these models to analyze patterns in real-world data is a difficult task due to intractability of likelihood-based inference. We propose applying Neural Posterior Estimation (NPE), a state-of-the-art approach to simulation-based inference, to perform efficient and accurate parameter estimation for complex infectious disease models. We will then demonstrate NPE’s ability to synthesize multiple datasets to infer individual-level patterns of transmission for a hospital-associated infection in a long-term acute care facility.