Mingyan Liu, PhD, is Professor of Electrical Engineering and Computer Science, College of Engineering, at the University of Michigan, Ann Arbor.
Prof. Liu’s research interest lies in optimal resource allocation, sequential decision theory, online and machine learning, performance modeling, analysis, and design of large-scale, decentralized, stochastic and networked systems, using tools including stochastic control, optimization, game theory and mechanism design. Her most recent research activities involve sequential learning, modeling and mining of large scale Internet measurement data concerning cyber security, and incentive mechanisms for inter-dependent security games. Within this context, her research group is actively working on the following directions.
1. Cyber security incident forecast. The goal is to predict an organization’s likelihood of having a cyber security incident in the near future using a variety of externally collected Internet measurement data, some of which capture active maliciousness (e.g., spam and phishing/malware activities) while others capture more latent factors (e.g., misconfiguration and mismanagement). While machine learning techniques have been extensively used for detection in the cyber security literature, using them for prediction has rarely been done. This is the first study on the prediction of broad categories of security incidents on an organizational level. Our work to date shows that with the right choice of feature set, highly accurate predictions can be achieved with a forecasting window of 6-12 months. Given the increasing amount of high profile security incidents (Target, Home Depot, JP Morgan Chase, and Anthem, just to name a few) and the amount of social and economic cost they inflict, this work will have a major impact on cyber security risk management.
2. Detect propagation in temporal data and its application to identifying phishing activities. Phishing activities propagate from one network to another in a highly regular fashion, a phenomenon known as fast-flux, though how the destination networks are chosen by the malicious campaign remains unknown. An interesting challenge arises as to whether one can use community detection methods to automatically extract those networks involved in a single phishing campaign; the ability to do so would be critical to forensic analysis. While there have been many results on detecting communities defined as subsets of relatively strongly connected entities, the phishing activity exhibits a unique propagating property that is better captured using an epidemic model. By using a combination of epidemic modeling and regression we can identify this type of propagating community with reasonable accuracy; we are working on alternative methods as well.
3. Data-driven modeling of organizational and end-user security posture. We are working to build models that accurately capture the cyber security postures of end-users as well as organizations, using large quantities of Internet measurement data. One domain is on how software vendors disclose security vulnerabilities in their products, how they deploy software upgrades and patches, and in turn, how end users install these patches; all these elements combined lead to a better understanding of the overall state of vulnerability of a given machine and how that relates to user behaviors. Another domain concerns the interconnectedness of today’s Internet which implies that what we see from one network is inevitably related to others. We use this connection to gain better insight into the conditions of not just a single network viewed in isolation, but multiple networks viewed together.
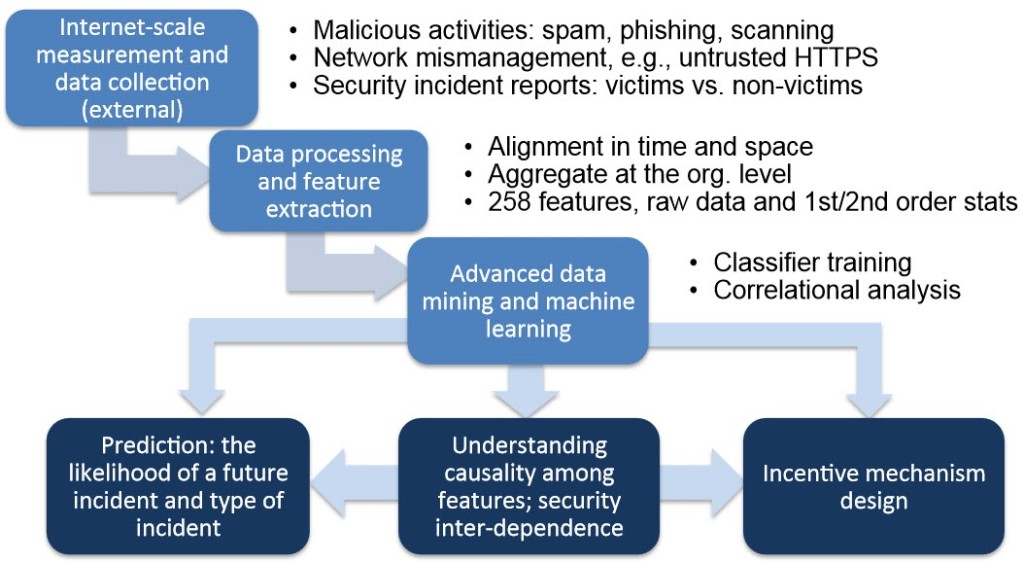