Professor Christopher Miller is an observational cosmologist who works in the fields of astronomical data mining and computational astrostatistics. He co-founded the INternational Computational Astrostatistics (INCA) group, a collaboration of researchers from the University of Michigan, Carnegie Mellon University, University of Washington, Georgia Tech, the NOAO, and others. Recently, he led the NOAO Science Data Management group, where he was responsible for using and delivering science quality astronomical data from instruments like the MOSAIC optical and NEWFIRM IR images on NOAO’s 4m-class telescopes. He was hired at the University of Michigan under a U-M Presidential initiative for advancing data mining research. His research and teaching emphasizes open source collaborative code development and the use of cloud computing to analyze large volumes of astronomical data. Professor Miller’s group develops and applies advanced computational and statistical techniques to address the following research areas:
- Cosmological parameter inference using the abundance and spatial distribution of clusters of galaxies.
- Evolution of the physical properties of the brightest galaxy cluster members.
- Morphological classification of galaxies.
- Dynamical techniques to trace the gravitational potential of galaxy clusters and probe the theory of gravity over large scales.
- Advanced data reduction pipelines for multi-object spectroscopic data.
- Concurrent real-world and simulated data analysis
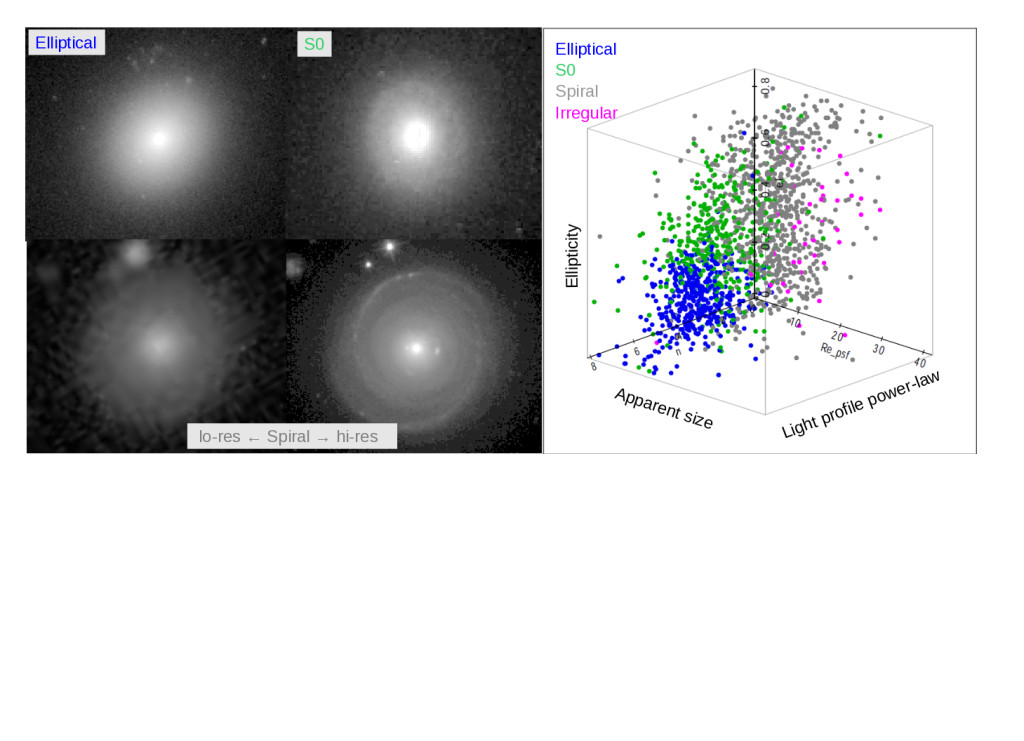