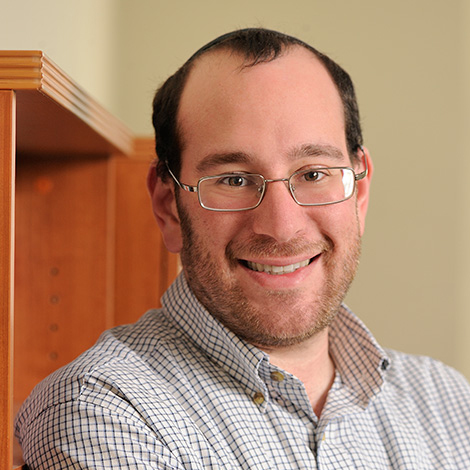
Large Language Models in Medicine: Opportunities and Challenges
Mark Dredze, John C Malone Professor of Computer Science; Director of Research (Foundations of AI), JHU AI-X Foundry; Johns Hopkins University
Abstract: The rapid advance of AI driven by Large Language Models (LLMs), like ChatGPT, has led to impressive results across a range of different use cases. This has included several models developed for the medical domain which have exhibited surprising behaviors, such as answering medical questions and performing well on medical licensing exams. These results have demonstrated the coming transformation of medicine by AI. In this talk, I will provide an overview of some of the recent advances in this area, and discuss challenges and opportunities for the use of these models in medicine.
Bio: Mark Dredze is the John C Malone Professor of Computer Science at Johns Hopkins University and the Director of Research (Foundations of AI) for the JHU AI-X Foundry. He develops Artificial Intelligence Systems based on natural language processing and explores applications to public health and medicine.
Prof. Dredze is affiliated with the Malone Center for Engineering in Healthcare, the Center for Language and Speech Processing, among others. He holds a joint appointment in the Biomedical Informatics & Data Science Section (BIDS), under the Department of Medicine (DOM), Division of General Internal Medicine (GIM) in the School of Medicine. He obtained his PhD from the University of Pennsylvania in 2009.
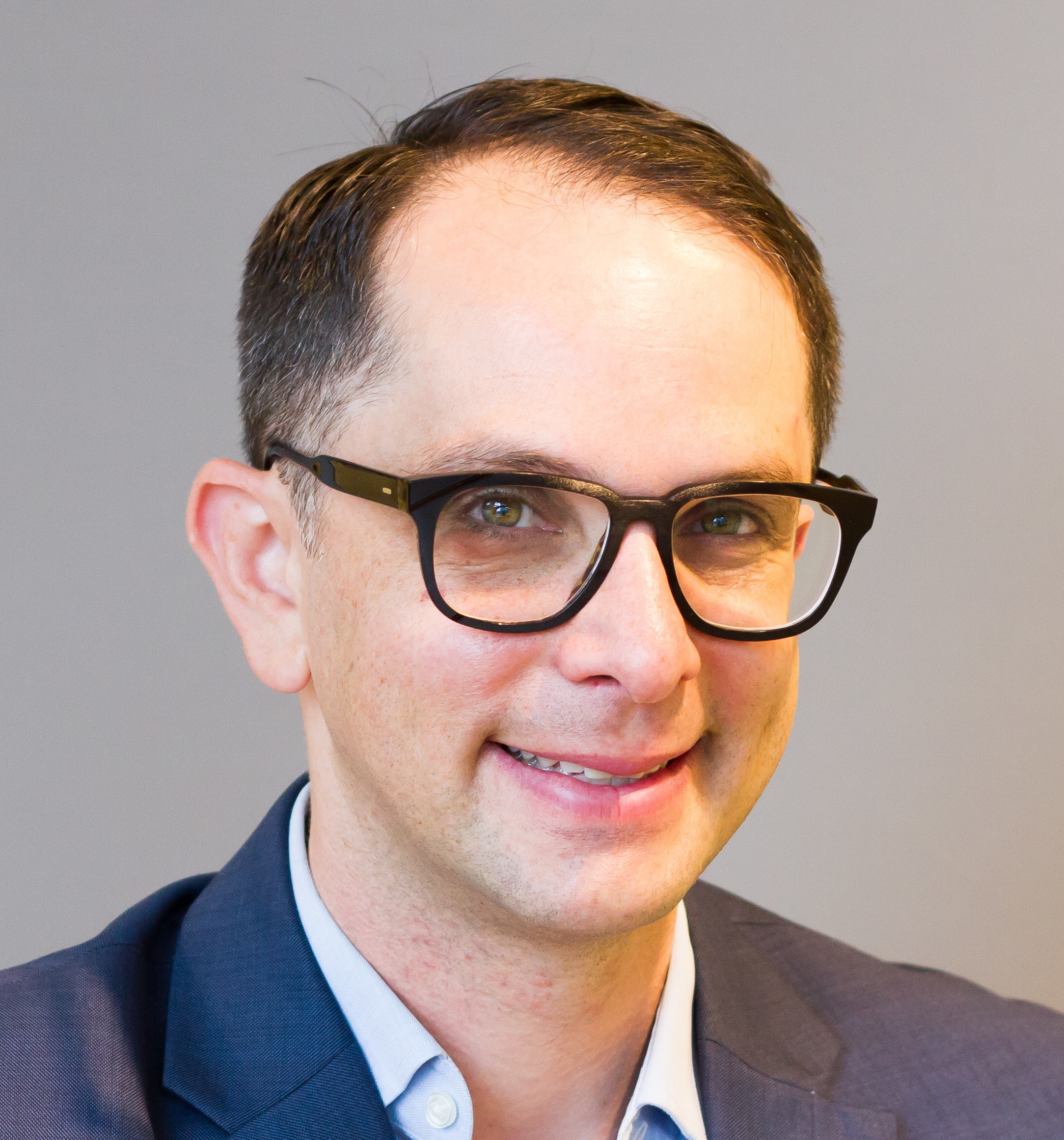
Generative AI in Global Health Metrics: opportunities and risks in natural language processing, AI-assisted data analysis, and simulation modeling
Abraham D. Flaxman, Associate Professor of Health Metrics Sciences, Institute for Health Metrics and Evaluation (IHME), University of Washington
View SlidesAbstract: Five years ago, I coauthored a short piece titled Machine learning in population health: Opportunities and threats. Now seems like an apt time to revisit it. We argued that AI could automate tasks that people do not like doing, cannot do fast enough, or cannot afford to do. The breakthroughs in generative AI over the last year have expanded the opportunities even further, but the threats have grown as well. We have very promising results from our preliminary investigations into the ability of large language models (LLMs) to identify underlying causes of death by analyzing so-called Verbal Autopsy Interviews, and I am optimistic about the potential for AI-assisted data analysis to broaden participation in technical analyses central to evidence-based public health. While I have only just begun to explore where generative AI fits into agent-based simulation modeling, it seems like a promising direction as well. However, the well-documented penchant of LLMs to hallucinate seems likely to amplify the challenges of explainability that have plagued nonparametric models in the past.
Bio: Abraham Flaxman, PhD, is an Associate Professor of Health Metrics Sciences at the Institute for Health Metrics and Evaluation (IHME) at the University of Washington. He is currently leading the development of a simulation platform to derive “what-if” results from Global Burden of Disease estimates and is engaged in methodological and operational research on verbal autopsy. Dr. Flaxman has previously designed software tools such as DisMod-MR that IHME uses to estimate the Global Burden of Disease, and the Bednet Stock-and-Flow Model, which has produced estimates of insecticide-treated net coverage in sub-Saharan Africa.
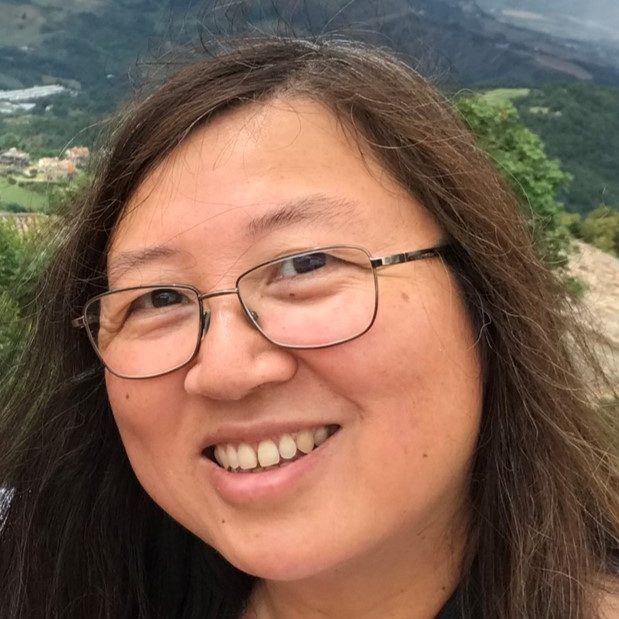
Moderator
Jing Liu, Executive Director, Michigan Institute for Data and AI in Society, University of Michigan