March 3rd, 2022 – MIDAS is excited to announce the 2021 Reproducibility Challenge winners and congratulates them for their outstanding work to make data science research more reproducible.
Jule Krüger (Center for Political Studies) and Ragnhild Nordås (Political Science), “Data Processing Principles for Auditable and Reproducible Research”.
Misha Teplitskiy (School of Information) and James Evans (Sociology, University of Chicago), “How Firm is Sociological Knowledge: Reanalysis of GSS findings with alternative models and out-of-sample data, 1972-2012”.
Yi Zhao (Biostatistics) and Xiaoquan Wen (Biostatistics), “Statistical Assessment of Replicability via Bayesian Model Criticism”.
A significant challenge across scientific fields is the reproducibility of research results, in both the narrow sense of repeating the calculations using the same data, and in the broader sense of deriving generalized insight from one or more investigations that bear on a common question. Ensuring that data science research results can be reliably reproduced is particularly challenging, especially as data science projects involve increasingly complex data and pipelines. In addition, when data science methodology is applied to vastly different research domains, the reproducibility of results hinges on domain-specific factors as well as components of the data science methodology.
The 2021 MIDAS Reproducibility Challenge winners bring to us principled yet creative work. Krüger and Nordås provide a template for a principled approach to organize, document and share a computational project. Teplitskiy and Evans use an automated and rigorous method to assess the reproducibility and replicability of survey data research and delineate causes for the lack of replicability.. Zhao and Wen propose a Bayesian model criticism approach to assess the replicability of a body of research studies that address the same scientific question with variations in data sets and analytical methods.
Through the 2020 and 2021 Reproducibility Challenges, MIDAS seeks exemplary work from our researchers to make data science projects more reproducible, and research outcomes more robust. We now have a wonderful collection of our researchers’ work to improve reproducibility in data science, and we are building collaboration with these researchers to enable the wide adoption of best practices.
Each of the winning teams will receive a cash prize. Later in the semester, they will present their work through a series of video showcases. Their projects will also be added to the MIDAS Reproducibility Hub.
Congratulations again to the winners.
Yi Zhao & Xiaoquan Wen
“Statistical Methods for Replicability Assessment”
Assessment of replicability is critical to ensure the quality and rigor of scientific research. In this work, we discuss inference and modeling principles for replicability assessment. Targeting distinct application scenarios, we propose two types of Bayesian model criticism approaches to identify potentially irreplicable results in scientific experiments. They are motivated by established Bayesian prior and posterior predictive model-checking procedures and generalize many existing replicability assessment methods. Finally, we discuss the statistical properties of the proposed replicability assessment approaches and illustrate their usages by an example of real data analysis, i.e., a systematic reanalysis of data from the Reproducibility Project: Psychology.
Jule Krüger & Ragnhild Nordås
“Data Processing Principles for Auditable and Reproducible Research”
In this project, we demonstrate a principled approach to data processing that allows researchers to automate their empirical research and make it scalable, auditable and reproducible. The approach comprises several principles: conceptualizing an empirical research project as tasks, organizing the project directory along these tasks, separating tasks into input files, source code, manual work and output files, using makefiles to automate and document the data workflow with regard to targets and dependencies, manually separating constants from code, adopting naming conventions that are self-explanatory, version controlling one’s work and publishing a public repository. Combined, these principles offer several benefits. They provide transparency about how the research data was manipulated either manually or computationally. They also quickly convey the role of each project file based on its name and location in the project directory and easily enable sensitivity analyses of modeling parameters and coding decisions. A practical example of this principled approach to data processing is available at https://github.com/juleka/JPR-LVM-SVAC.
Misha Teplitskiy & James Evans
“How Firm is Sociological Knowledge?: Reanalysis of General Social Survey findings with alternative models of out-of-sample data”
This project proposes a method to empirically measure the replicability of social science literatures that rely on survey data. Published findings in such literatures may be fragile because hypotheses were tailored to fit the data (“data mining” or “p- hacking”) or because the world has changed and once robust relationships no longer hold (“social change”). The proposed method measures failure to replicate due to both causes. To exemplify the method, we apply it to findings from hundreds of publications that use the General Social Survey, 1972 – 2012. To measure the consequences of data mining, we estimate the published regression models on the original data and compare them to alternative specifications on the same data. To measure the consequences of social change, we estimate the published models on the original data and compare them to the same models estimated on subsequent waves of the GSS, which came out after publication. There is evidence that both mechanisms decrease replicability: the number of significant coefficients, standardized coefficient sizes, and R2 are significantly reduced in both analyses. However, the reduction in significance levels and effect sizes is larger for the social change mechanism. Our findings suggest that social scientists are engaged in only moderate data mining; a bigger concern is the decreased relevance of older published knowledge to the contemporary world.
The MIDAS Reproducibility Challenge II is open to researchers from any field that makes use of data, broadly construed. We seek entries in four categories:
Training materials for reproducible research. These should be well developed materials for courses or workshops, with a clear theme that can be easily adopted by other instructors.
Guides, processes and tools that can be readily adopted by researchers in one or multiple research areas. Other researchers, even if only within a narrowly defined research field, should be able to follow such guides, processes and tools to make their work reproducible.
A template for a reproducible project. The goal is to allow others to emulate and develop similar approaches and lower the burden of making their projects reproducible. We especially welcome submissions that seek to make analyses not only reproducible, but transparent. This might include especially well organized or well documented code (for example, using notebooks); saving intermediate datasets / results so that they can be easily inspected; code that draws attention to and allows easy modification of analytical choices such as statistical tuning parameters, data cleaning choices, etc.
An analysis of reproducibility in a research field, including meta-analysis or reproducing published works, to identify good practices for and challenges to replication and confirmation of published works, and proposals for new practices and tools to overcome barriers. A research field needs to be well defined, but can be broad or narrow, e.g. “single-cell RNAseq”, or “child development research with video data”.
Planning Committee
George Alter
Research Professor, ICPSR
Professor of History
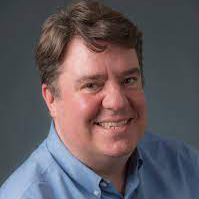
Jacob Carlson
Director of Deep Blue Repository and Research Data Services, U-M
Johann Gagnon Bartsch
Assistant Professor, Statistics
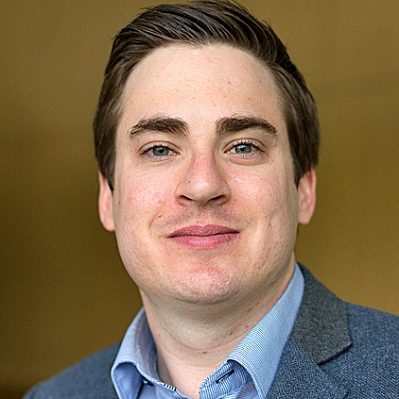
Bryan Goldsmith
Assistant Professor, Chemical Engineering
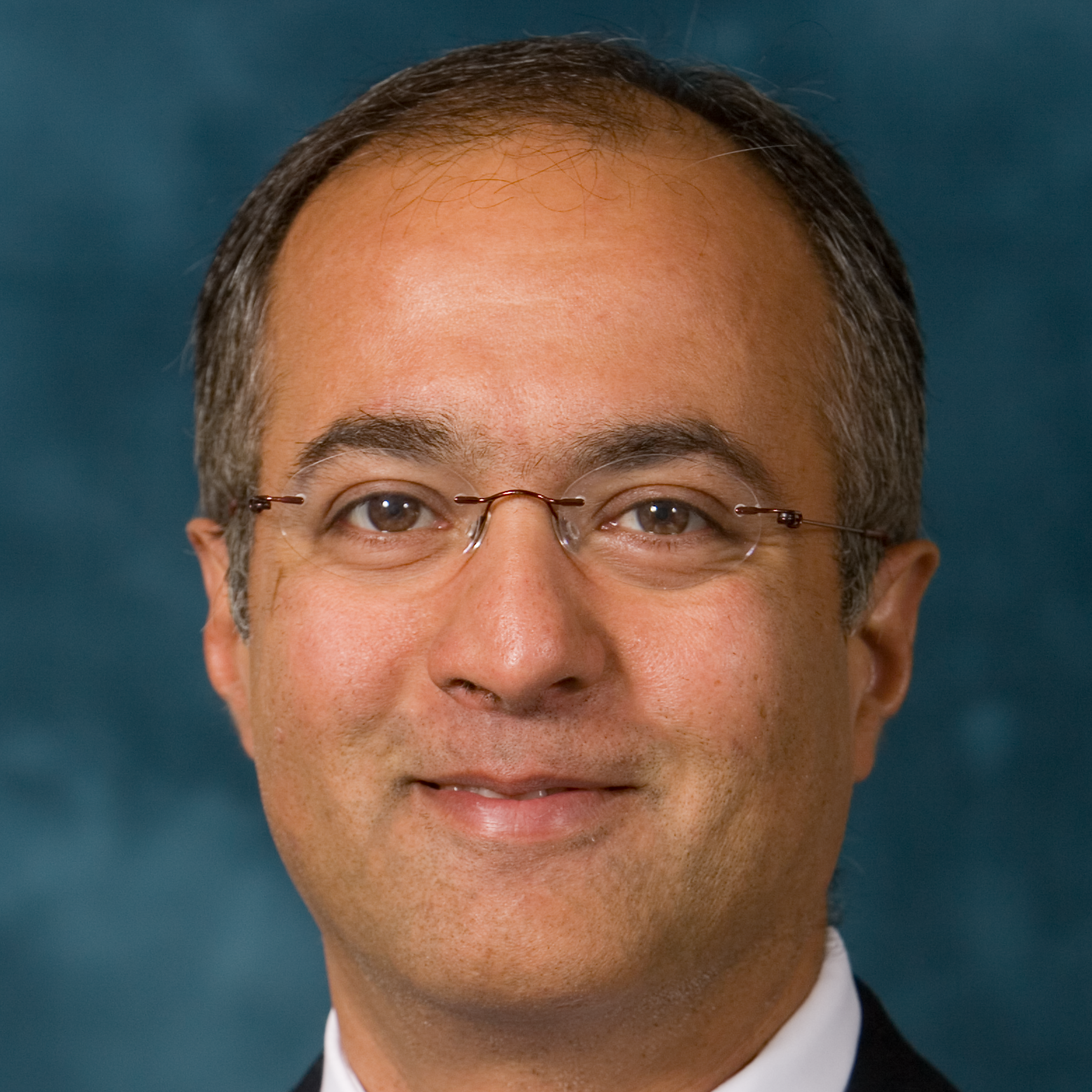
H.V. Jagadish
MIDAS Director
Professor, Electrical Engineering and Computer Science

Jing Liu
Managing Director, MIDAS
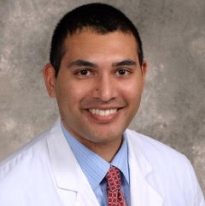
Thomas Valley
Assistant Professor, U-M Department of Internal Medicine